Published:
May 2004
Proceedings:
Proceedings of the Seventeenth International Florida Artificial Intelligence Research Society Conference (FLAIRS 2004)
Volume
Issue:
Proceedings of the Seventeenth International Florida Artificial Intelligence Research Society Conference (FLAIRS 2004)
Track:
All Papers
Downloads:
Abstract:
In the neural network literature, input feature de-correlation is often referred as one pre-processing technique used to improve the MLP training speed. However, in this paper, we find that de-correlation by orthogonal Karhunen-Loeve transform (KLT) may not be helpful to improve training. Through detailed analyses, the effect of input de-correlation is revealed to be equivalent to using a different weight set to initialize the network. Thus, for a robust training algorithm, the benefit of input de-correlation would be negligible. The theoretical results are applicable to several gradient training algorithms, i.e. back-propagation, conjugate gradient. The simulation results confirm our theoretical analyses.
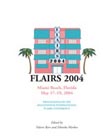
FLAIRS
Proceedings of the Seventeenth International Florida Artificial Intelligence Research Society Conference (FLAIRS 2004)
ISBN 978-1-57735-201-3
Published by The AAAI Press, Menlo Park, California.