Published:
May 2000
Proceedings:
Proceedings of the Thirteenth International Florida Artificial Intelligence Research Society Conference (FLAIRS 2000)
Volume
Issue:
Proceedings of the Thirteenth International Florida Artificial Intelligence Research Society Conference (FLAIRS 2000)
Track:
All Papers
Downloads:
Abstract:
We proposea scheme for producing Latin hypercube samples that can enhance any of the existing sampling algorithms in Bayesian networks. We test this scheme in combination with the likelihood weighting algorithm and show that it can lead to a significant improvement in the convergence rate. While performance of sampling algorithms in general depends on the numerical properties of a network, in our experiments Latin hypercube sampling performed always better than random sampling. In some cases we observed as much as an order of magnitude improvement in convergence rates. We discuss practical issues related to storage requirements of Latin hypercube sample generation and propose a low-storage, anytime cascaded version of Latin hypercube sampling that introduces a minimal performance loss compared to the original scheme.
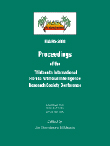
FLAIRS
Proceedings of the Thirteenth International Florida Artificial Intelligence Research Society Conference (FLAIRS 2000)
ISBN 978-1-57735-113-9
Published by The AAAI Press, Menlo Park, California.