Published:
May 2000
Proceedings:
Proceedings of the Thirteenth International Florida Artificial Intelligence Research Society Conference (FLAIRS 2000)
Volume
Issue:
Proceedings of the Thirteenth International Florida Artificial Intelligence Research Society Conference (FLAIRS 2000)
Track:
All Papers
Downloads:
Abstract:
In response to the difficulties traditionally experience in knowledge acquisition for knowledge-based systems, we wish to allow domain experts to work directly with the knowledge base in a more intuitive manner. However, we also wish to represent uncertainty in our knowledge. Unfortunately, knowledge representations that handle uncertainty are counter intuitive and difficult to use. To achieve these dual goals, we introduce a layer of abstraction above the underlying knowledge representation. Probabilistic formalisms are preferred because they provide a means for modeling uncertainty in the rules by weighing our confidence in a conclusion with a probability value. The abstraction allows the expert user to specify knowledge in familiar if-then style rules in forms that appear often and are difficult to construct with the existing knowledge representation rules. For example, we may desire to weigh our confidence in a conclusion based on the number of pieces of evidence found in agreement with that conclusion. Given only the evidence and a conclusion from the user, we wish for the system to construct the corresponding rule in the knowledge representation automatically, leaving the implementation details hidden from the domain expert. With these rules built automatically by the system, the user can concentrate on expressing the domain knowledge rather than the structure and consistency of the knowledge base.
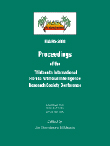
FLAIRS
Proceedings of the Thirteenth International Florida Artificial Intelligence Research Society Conference (FLAIRS 2000)
ISBN 978-1-57735-113-9
Published by The AAAI Press, Menlo Park, California.