Published:
May 2000
Proceedings:
Proceedings of the Thirteenth International Florida Artificial Intelligence Research Society Conference (FLAIRS 2000)
Volume
Issue:
Proceedings of the Thirteenth International Florida Artificial Intelligence Research Society Conference (FLAIRS 2000)
Track:
All Papers
Downloads:
Abstract:
Highly optimized decision trees which have been created from ID3-type algorithms are often recognized as being one of the best methods for partitioning a given domain in terms of both classification accuracy and for the formulation of small rule sets. However, these trees are highly optimized and potential information in lower branches of the tree is lost through pruning. This paper presents a novel algorithm that regains this information by relazing sharp decition boundaries and by regrowing the decision tree by relaxing the pruning criteria.
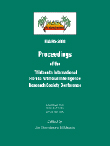
FLAIRS
Proceedings of the Thirteenth International Florida Artificial Intelligence Research Society Conference (FLAIRS 2000)
ISBN 978-1-57735-113-9
Published by The AAAI Press, Menlo Park, California.