Published:
May 1999
Proceedings:
Proceedings of the Twelfth International Florida Artificial Intelligence Research Society Conference (FLAIRS 1999)
Volume
Issue:
Proceedings of the Twelfth International Florida Artificial Intelligence Research Society Conference (FLAIRS 1999)
Track:
All Papers
Downloads:
Abstract:
We report preliminary results on a weighted instance-based algorithm for the problem of autonomous robot learning. The algorithm combines the K-nearest neighbour technique and a distance metric which provides selective spreading of learning updates on the experience space, with the aim of minimimizing the problem of partial state observability produced by local sensor readings and insufficient global information. Results show that the algorithm generated a good action policy for a simulated guidance robot with two basic behaviours (preprogrammed obstacle avoidance and learned target approximation).
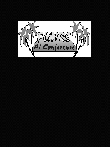
FLAIRS
Proceedings of the Twelfth International Florida Artificial Intelligence Research Society Conference (FLAIRS 1999)
ISBN 978-1-57735-080-4
Published by The AAAI Press, Menlo Park, California.