Published:
May 1999
Proceedings:
Proceedings of the Twelfth International Florida Artificial Intelligence Research Society Conference (FLAIRS 1999)
Volume
Issue:
Proceedings of the Twelfth International Florida Artificial Intelligence Research Society Conference (FLAIRS 1999)
Track:
All Papers
Downloads:
Abstract:
We study data mining where the task is description by summarization, the representation language is generalized relations, the evaluation criteria are based on heuristic measures of interestingness, and the method for searching is the Multi-Attribute Generalization algorithm for domain generalization graphs. We present and empirically compare four heuristics for ranking the interestingness of generalized relations (or summaries). The measures are based on common measures of the diversity of a population, statistical variance, the Simpson index, and the Shannon index. All four measures rank less complex summaries (i.e., those with few tuples and/or non-ANY attributes) as most interesting. Highly ranked summaries provide a reasonable starting point for further analysis of discovered knowledge.
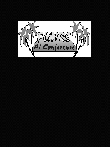
FLAIRS
Proceedings of the Twelfth International Florida Artificial Intelligence Research Society Conference (FLAIRS 1999)
ISBN 978-1-57735-080-4
Published by The AAAI Press, Menlo Park, California.