Published:
2020-06-02
Proceedings:
Proceedings of the AAAI Conference on Artificial Intelligence, 34
Volume
Issue:
Vol. 34 No. 10: Issue 10: AAAI-20 Student Tracks
Track:
Student Abstract Track
Downloads:
Abstract:
We demonstrate that projecting data points into hyperplanes is good strategy for general-purpose kernel design. We used three different hyperplanes generation schemes, random, convex hull and α-shape, and evaluated the results on two synthetic and three well known image-based datasets. The results showed considerable improvement in the classification performance in almost all scenarios, corroborating the claim that such an approach can be used as a general-purpose kernel transformation. Also, we discuss some connection with Convolutional Neural Networks and how such an approach could be used to understand such networks better.
DOI:
10.1609/aaai.v34i10.7211
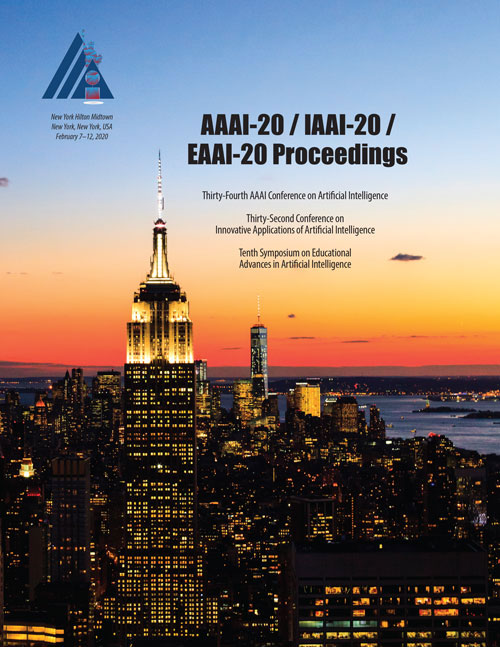
AAAI
Vol. 34 No. 10: Issue 10: AAAI-20 Student Tracks
ISSN 2374-3468 (Online) ISSN 2159-5399 (Print) ISBN 978-1-57735-835-0 (10 issue set)
Published by AAAI Press, Palo Alto, California USA Copyright © 2020, Association for the Advancement of Artificial Intelligence All Rights Reserved