Published:
2018-02-08
Proceedings:
Proceedings of the AAAI Conference on Artificial Intelligence, 32
Volume
Issue:
Thirty-Second AAAI Conference on Artificial Intelligence 2018
Track:
AAAI Technical Track: Vision
Downloads:
Abstract:
Multi-view based shape descriptors have achieved impressive performance for 3D shape retrieval. The core of view-based methods is to interpret 3D structures through 2D observations. However, most existing methods pay more attention to discriminative models and none of them necessarily incorporate the 3D properties of the objects. To resolve this problem, we propose an encoder-decoder recurrent feature aggregation network (ERFA-Net) to emphasize the 3D properties of 3D shapes in multi-view features aggregation. In our network, a view sequence of the shape is trained to encode a discriminative shape embedding and estimate unseen rendered views of any viewpoints. This generation task gives an effective supervision which makes the network exploit 3D properties of shapes through various 2D images. During feature aggregation, a discriminative feature representation across multiple views is effectively exploited based on LSTM network. The proposed 3D representation has following advantages against other state-of-the-art: 1) it performs robust discrimination under the existence of noise such as view missing and occlusion, because of the improvement brought by 3D properties. 2) it has strong generative capabilities, which is useful for various 3D shape tasks. We evaluate ERFA-Net on two popular 3D shape datasets, ModelNet and ShapeNetCore55, and ERFA-Net outperforms the state-of-the-art methods significantly. Extensive experiments show the effectiveness and robustness of the proposed 3D representation.
DOI:
10.1609/aaai.v32i1.12309
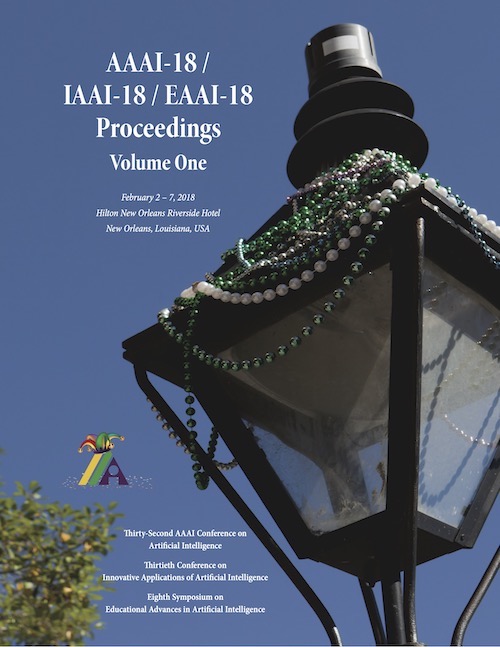
AAAI
Thirty-Second AAAI Conference on Artificial Intelligence 2018
ISSN 2374-3468 (Online) ISSN 2159-5399 (Print)
Published by AAAI Press, Palo Alto, California USA Copyright © 2018, Association for the Advancement of Artificial Intelligence All Rights Reserved.