Published:
2018-02-08
Proceedings:
Proceedings of the AAAI Conference on Artificial Intelligence, 32
Volume
Issue:
Thirty-Second AAAI Conference on Artificial Intelligence 2018
Track:
AAAI Technical Track: Vision
Downloads:
Abstract:
Zero-shot learning (ZSL) is regarded as an effective way to construct classification models for target classes which have no labeled samples available. The basic framework is to transfer knowledge from (different) auxiliary source classes having sufficient labeled samples with some attributes shared by target and source classes as bridge. Attributes play an important role in ZSL but they have not gained sufficient attention in recent years. Previous works mostly assume attributes are perfect and treat each attribute equally. However, as shown in this paper, different attributes have different properties, such as their class distribution, variance, and entropy, which may have considerable impact on ZSL accuracy if treated equally. Based on this observation, in this paper we propose to use a subset of attributes, instead of the whole set, for building ZSL models. The attribute selection is conducted by considering the information amount and predictability under a novel joint optimization framework. To our knowledge, this is the first work that notices the influence of attributes themselves and proposes to use a refined attribute set for ZSL. Since our approach focuses on selecting good attributes for ZSL, it can be combined to any attribute based ZSL approaches so as to augment their performance. Experiments on four ZSL benchmarks demonstrate that our approach can improve zero-shot classification accuracy and yield state-of-the-art results.
DOI:
10.1609/aaai.v32i1.12251
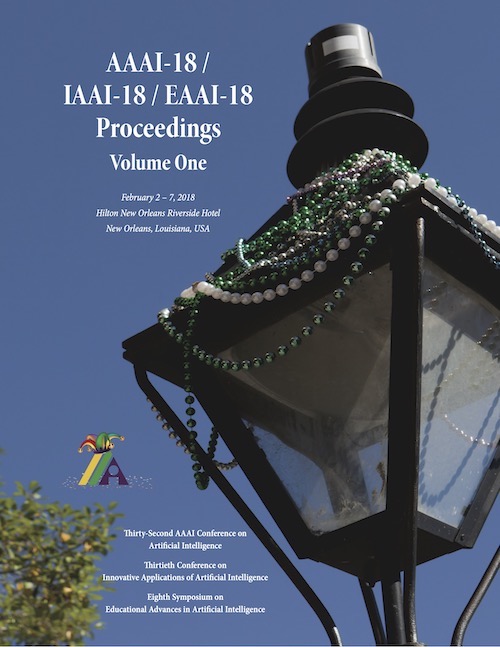
AAAI
Thirty-Second AAAI Conference on Artificial Intelligence 2018
ISSN 2374-3468 (Online) ISSN 2159-5399 (Print)
Published by AAAI Press, Palo Alto, California USA Copyright © 2018, Association for the Advancement of Artificial Intelligence All Rights Reserved.