Published:
2018-02-08
Proceedings:
Proceedings of the AAAI Conference on Artificial Intelligence, 32
Volume
Issue:
Thirty-Second AAAI Conference on Artificial Intelligence 2018
Track:
Student Abstract Track
Downloads:
Abstract:
Many real-life problems require optimizing functions with expensive evaluations. Bayesian Optimization (BO) and Search-based Optimization (SO) are two broad families of algorithms that try to find the global optima of a function with the goal of minimizing the number of function evaluations. A large body of existing work deals with the single-fidelity setting, where function evaluations are very expensive but accurate. However, in many applications, we have access to multiple-fidelity functions that vary in their cost and accuracy of evaluation. In this paper, we propose a novel approach called Multi-fidelity Hybrid (MF-Hybrid) that combines the best attributes of both BO and SO methods to discover the global optima of a black-box function with minimal cost. Our experiments on multiple benchmark functions show that the MF-Hybrid algorithm outperforms existing single-fidelity and multi-fidelity optimization algorithms.
DOI:
10.1609/aaai.v32i1.12184
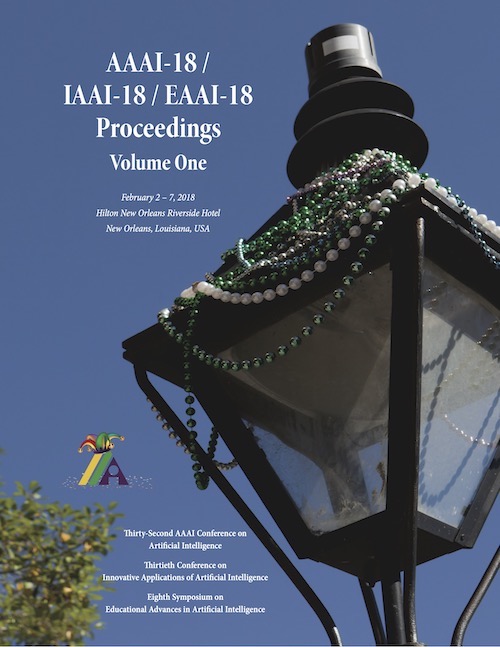
AAAI
Thirty-Second AAAI Conference on Artificial Intelligence 2018
ISSN 2374-3468 (Online) ISSN 2159-5399 (Print)
Published by AAAI Press, Palo Alto, California USA Copyright © 2018, Association for the Advancement of Artificial Intelligence All Rights Reserved.