Published:
2020-06-02
Proceedings:
Proceedings of the AAAI Conference on Artificial Intelligence, 34
Volume
Issue:
Vol. 34 No. 07: AAAI-20 Technical Tracks 7
Track:
AAAI Technical Track: Vision
Downloads:
Abstract:
In this paper, we propose an improved end-to-end multi-branch person search network to jointly optimize person detection, re-identification, instance segmentation, and keypoint detection. First, we build a better and faster base model to extract non-highly correlated feature expression; Second, a foreground feature enhance module is used to alleviate undesirable background noise in person feature maps; Third, we design an algorithm to learn the part-aligned representation for person search. Extensive experiments with ablation analysis show the effectiveness of our proposed end-to-end multi-task model, and we demonstrate its superiority over the state-of-the-art methods on two benchmark datasets including CUHK-SYSU and PRW.
DOI:
10.1609/aaai.v34i07.6886
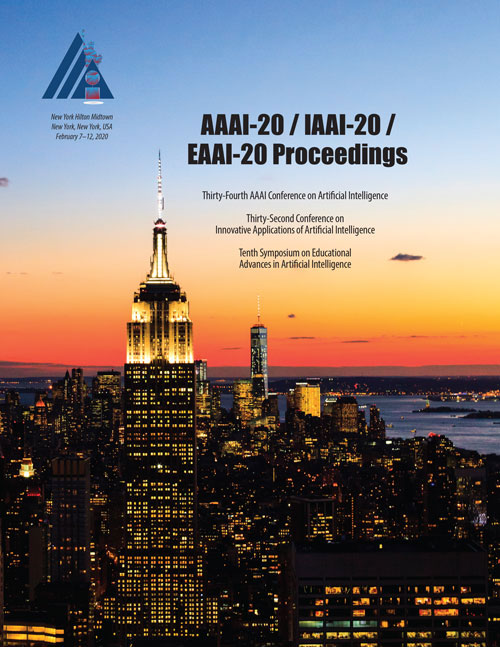
AAAI
Vol. 34 No. 07: AAAI-20 Technical Tracks 7
ISSN 2374-3468 (Online) ISSN 2159-5399 (Print) ISBN 978-1-57735-835-0 (10 issue set)
Published by AAAI Press, Palo Alto, California USA Copyright © 2020, Association for the Advancement of Artificial Intelligence All Rights Reserved