Published:
2018-02-08
Proceedings:
Proceedings of the AAAI Conference on Artificial Intelligence, 32
Volume
Issue:
Thirty-Second AAAI Conference on Artificial Intelligence 2018
Track:
Main Track: NLP and Knowledge Representation
Downloads:
Abstract:
Identifying event instance in text plays a critical role in building NLP applications such as Information Extraction (IE) system. However, most existing methods for this task focus only on monolingual clues of a specific language and ignore the massive information provided by other languages. Data scarcity and monolingual ambiguity hinder the performance of these monolingual approaches. In this paper, we propose a novel multilingual approach---dubbed as Gated Multilingual Attention (GMLATT) framework---to address the two issues simultaneously. In specific, to alleviate data scarcity problem, we exploit the consistent information in multilingual data via context attention mechanism. Which takes advantage of the consistent evidence in multilingual data other than learning only from monolingual data. To deal with monolingual ambiguity problem, we propose gated cross-lingual attention to exploit the complement information conveyed by multilingual data, which is helpful for the disambiguation. The cross-lingual attention gate serves as a sentinel modelling the confidence of the clues provided by other languages and controls the information integration of various languages. We have conducted extensive experiments on the ACE 2005 benchmark. Experimental results show that our approach significantly outperforms state-of-the-art methods.
DOI:
10.1609/aaai.v32i1.11919
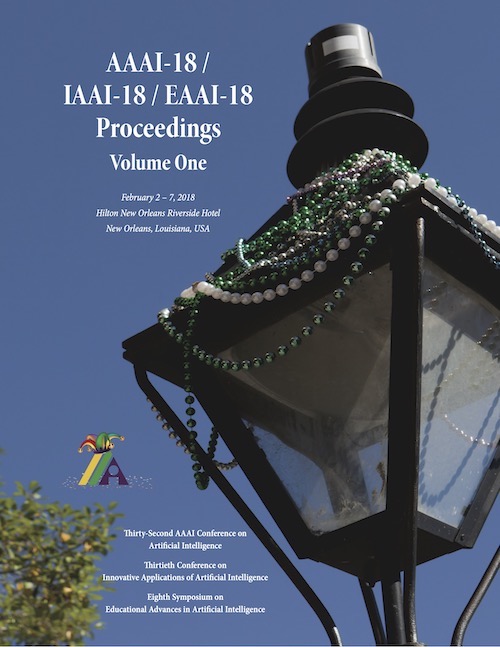
AAAI
Thirty-Second AAAI Conference on Artificial Intelligence 2018
ISSN 2374-3468 (Online) ISSN 2159-5399 (Print)
Published by AAAI Press, Palo Alto, California USA Copyright © 2018, Association for the Advancement of Artificial Intelligence All Rights Reserved.