Published:
2018-02-08
Proceedings:
Proceedings of the AAAI Conference on Artificial Intelligence, 32
Volume
Issue:
Thirty-Second AAAI Conference on Artificial Intelligence 2018
Track:
Main Track: Machine Learning Applications
Downloads:
Abstract:
Understanding politics is challenging because the politics take the influence from everything. Even we limit ourselves to the political context in the legislative processes; we need a better understanding of latent factors, such as legislators, bills, their ideal points, and their relations. From the modeling perspective, this is difficult 1) because these observations lie in a high dimension that requires learning on low dimensional representations, and 2) because these observations require complex probabilistic modeling with latent variables to reflect the causalities. This paper presents a new model to reflect and understand this political setting, NIPEN, including factors mentioned above in the legislation. We propose two versions of NIPEN: one is a hybrid model of deep learning and probabilistic graphical model, and the other model is a neural tensor model. Our result indicates that NIPEN successfully learns the manifold of the legislative bill's text, and NIPEN utilizes the learned low-dimensional latent variables to increase the prediction performance of legislators' votings. Additionally, by virtue of being a domain-rich probabilistic model, NIPEN shows the hidden strength of the legislators' trust network and their various characteristics on casting votes.
DOI:
10.1609/aaai.v32i1.11852
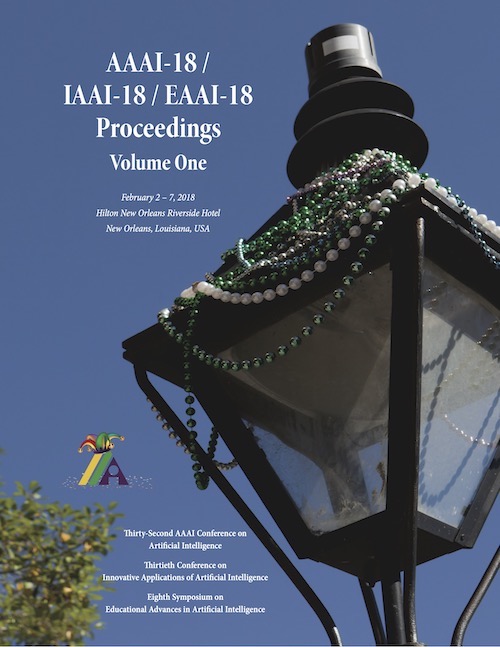
AAAI
Thirty-Second AAAI Conference on Artificial Intelligence 2018
ISSN 2374-3468 (Online) ISSN 2159-5399 (Print)
Published by AAAI Press, Palo Alto, California USA Copyright © 2018, Association for the Advancement of Artificial Intelligence All Rights Reserved.