Published:
2018-02-08
Proceedings:
Proceedings of the AAAI Conference on Artificial Intelligence, 32
Volume
Issue:
Thirty-Second AAAI Conference on Artificial Intelligence 2018
Track:
AAAI Technical Track: Machine Learning
Downloads:
Abstract:
Humans and animals learn much better when the examples are not randomly presented but organized in a meaningful order which illustrates gradually more concepts, and gradually more complex ones. Inspired by this curriculum learning mechanism, we propose a reinforced multi-label image classification approach imitating human behavior to label image from easy to complex. This approach allows a reinforcement learning agent to sequentially predict labels by fully exploiting image feature and previously predicted labels. The agent discovers the optimal policies through maximizing the long-term reward which reflects prediction accuracies. Experimental results on PASCAL VOC2007 and 2012 demonstrate the necessity of reinforcement multi-label learning and the algorithm’s effectiveness in real-world multi-label image classification tasks.
DOI:
10.1609/aaai.v32i1.11770
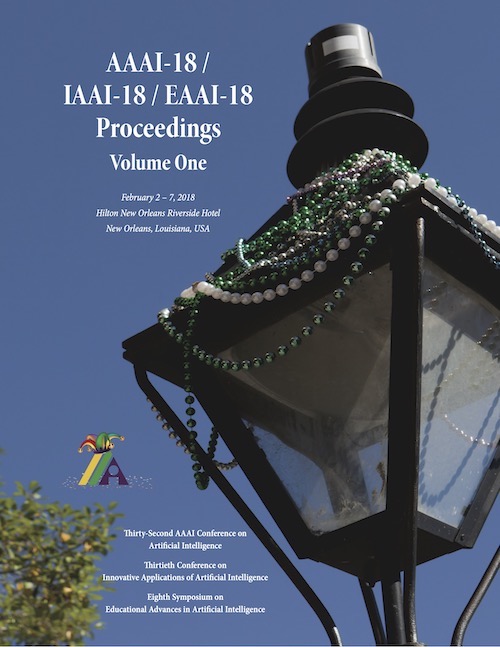
AAAI
Thirty-Second AAAI Conference on Artificial Intelligence 2018
ISSN 2374-3468 (Online) ISSN 2159-5399 (Print)
Published by AAAI Press, Palo Alto, California USA Copyright © 2018, Association for the Advancement of Artificial Intelligence All Rights Reserved.