Published:
2020-06-02
Proceedings:
Proceedings of the AAAI Conference on Artificial Intelligence, 34
Volume
Issue:
Vol. 34 No. 05: AAAI-20 Technical Tracks 5
Track:
AAAI Technical Track: Natural Language Processing
Downloads:
Abstract:
Dirichlet-multinomial (D-M) mixtures like latent Dirichlet allocation (LDA) are widely used for both topic modeling and clustering. Prior work on constructing Levin-style semantic verb clusters achieves state-of-the-art results using D-M mixtures for verb sense induction and clustering. We add a bias toward known clusters by explicitly labeling a small number of observations with their correct VerbNet class. We demonstrate that this partial supervision guides the resulting clusters effectively, improving the recovery of both labeled and unlabeled classes by 16%, for a joint 12% absolute improvement in F1 score compared to clustering without supervision. The resulting clusters are also more semantically coherent. Although the technical change is minor, it produces a large effect, with important practical consequences for supervised topic modeling in general.
DOI:
10.1609/aaai.v34i05.6385
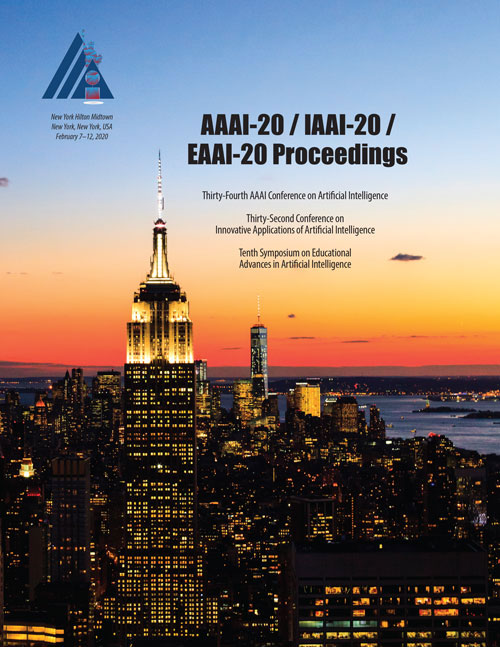
AAAI
Vol. 34 No. 05: AAAI-20 Technical Tracks 5
ISSN 2374-3468 (Online) ISSN 2159-5399 (Print) ISBN 978-1-57735-835-0 (10 issue set)
Published by AAAI Press, Palo Alto, California USA Copyright © 2020, Association for the Advancement of Artificial Intelligence All Rights Reserved