Published:
2020-06-02
Proceedings:
Proceedings of the AAAI Conference on Artificial Intelligence, 34
Volume
Issue:
Vol. 34 No. 05: AAAI-20 Technical Tracks 5
Track:
AAAI Technical Track: Natural Language Processing
Downloads:
Abstract:
Taking an answer and its context as input, sequence-to-sequence models have made considerable progress on question generation. However, we observe that these approaches often generate wrong question words or keywords and copy answer-irrelevant words from the input. We believe that lacking global question semantics and exploiting answer position-awareness not well are the key root causes. In this paper, we propose a neural question generation model with two general modules: sentence-level semantic matching and answer position inferring. Further, we enhance the initial state of the decoder by leveraging the answer-aware gated fusion mechanism. Experimental results demonstrate that our model outperforms the state-of-the-art (SOTA) models on SQuAD and MARCO datasets. Owing to its generality, our work also improves the existing models significantly.
DOI:
10.1609/aaai.v34i05.6366
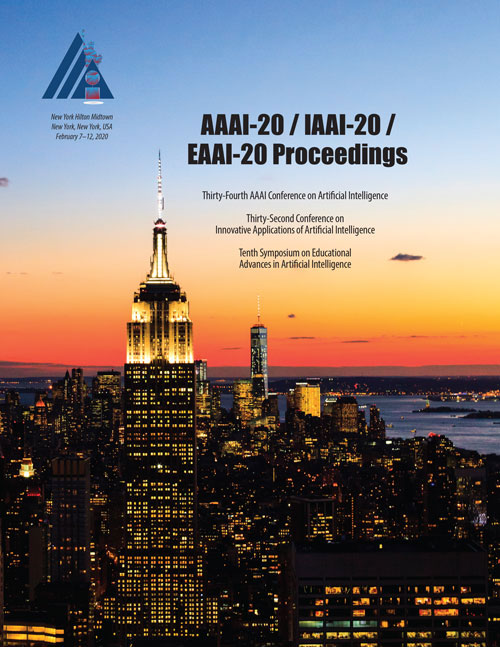
AAAI
Vol. 34 No. 05: AAAI-20 Technical Tracks 5
ISSN 2374-3468 (Online) ISSN 2159-5399 (Print) ISBN 978-1-57735-835-0 (10 issue set)
Published by AAAI Press, Palo Alto, California USA Copyright © 2020, Association for the Advancement of Artificial Intelligence All Rights Reserved