Published:
2020-06-02
Proceedings:
Proceedings of the AAAI Conference on Artificial Intelligence, 34
Volume
Issue:
Vol. 34 No. 05: AAAI-20 Technical Tracks 5
Track:
AAAI Technical Track: Natural Language Processing
Downloads:
Abstract:
We propose to cast the task of morphological inflection—mapping a lemma to an indicated inflected form—for resource-poor languages as a meta-learning problem. Treating each language as a separate task, we use data from high-resource source languages to learn a set of model parameters that can serve as a strong initialization point for fine-tuning on a resource-poor target language. Experiments with two model architectures on 29 target languages from 3 families show that our suggested approach outperforms all baselines. In particular, it obtains a 31.7% higher absolute accuracy than a previously proposed cross-lingual transfer model and outperforms the previous state of the art by 1.7% absolute accuracy on average over languages.
DOI:
10.1609/aaai.v34i05.6316
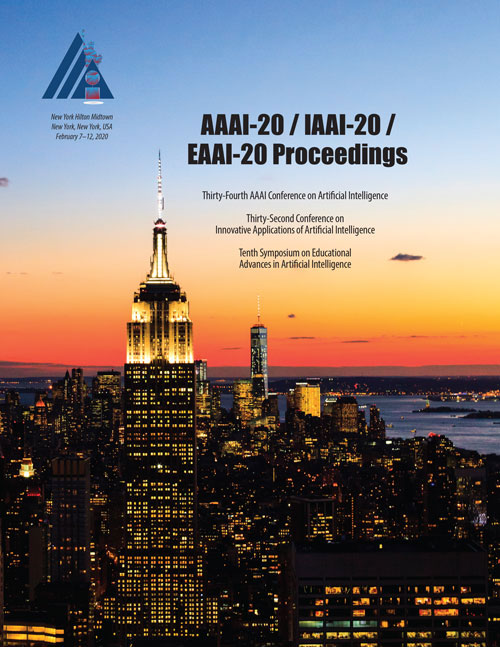
AAAI
Vol. 34 No. 05: AAAI-20 Technical Tracks 5
ISSN 2374-3468 (Online) ISSN 2159-5399 (Print) ISBN 978-1-57735-835-0 (10 issue set)
Published by AAAI Press, Palo Alto, California USA Copyright © 2020, Association for the Advancement of Artificial Intelligence All Rights Reserved