Published:
2020-06-02
Proceedings:
Proceedings of the AAAI Conference on Artificial Intelligence, 34
Volume
Issue:
Vol. 34 No. 04: AAAI-20 Technical Tracks 4
Track:
AAAI Technical Track: Machine Learning
Downloads:
Abstract:
Multi-view spectral clustering aims to group data into different categories by optimally exploring complementary information from multiple Laplacian matrices. However, existing methods usually linearly combine a group of pre-specified first-order Laplacian matrices to construct an optimal Laplacian matrix, which may result in limited representation capability and insufficient information exploitation. In this paper, we propose a novel optimal neighborhood multi-view spectral clustering (ONMSC) algorithm to address these issues. Specifically, the proposed algorithm generates an optimal Laplacian matrix by searching the neighborhood of both the linear combination of the first-order and high-order base Laplacian matrices simultaneously. This design enhances the representative capacity of the optimal Laplacian and better utilizes the hidden high-order connection information, leading to improved clustering performance. An efficient algorithm with proved convergence is designed to solve the resultant optimization problem. Extensive experimental results on 9 datasets demonstrate the superiority of our algorithm against state-of-the-art methods, which verifies the effectiveness and advantages of the proposed ONMSC.
DOI:
10.1609/aaai.v34i04.6180
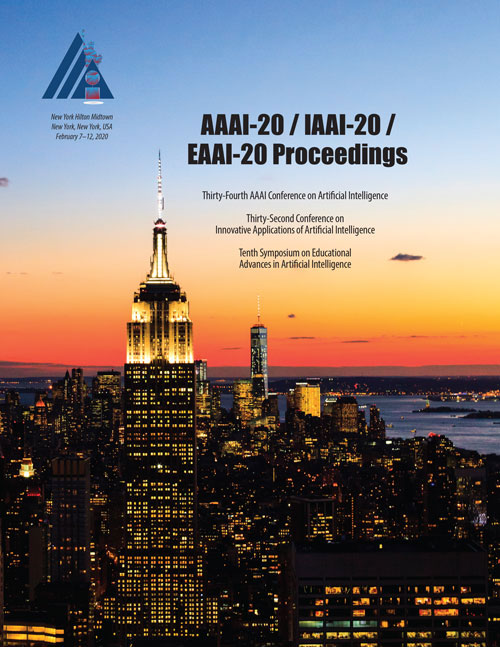
AAAI
Vol. 34 No. 04: AAAI-20 Technical Tracks 4
ISSN 2374-3468 (Online) ISSN 2159-5399 (Print) ISBN 978-1-57735-835-0 (10 issue set)
Published by AAAI Press, Palo Alto, California USA Copyright © 2020, Association for the Advancement of Artificial Intelligence All Rights Reserved