Published:
2016-11-03
Proceedings:
Proceedings of the AAAI Conference on Human Computation and Crowdsourcing, 4
Volume
Issue:
Vol. 4 (2016): Fourth AAAI Conference on Human Computation and Crowdsourcing
Track:
Full Papers
Downloads:
Abstract:
Crowd workers are human and thus sometimes make mistakes. In order to ensure the highest quality output, requesters often issue redundant jobs with gold test questions and sophisticated aggregation mechanisms based on expectation maximization (EM). While these methods yield accurate results in many cases, they fail on extremely difficult problems with local minima, such as situations where the majority of workers get the answer wrong. Indeed, this has caused some researchers to conclude that on some tasks crowdsourcing can never achieve high accuracies, no matter how many workers are involved. This paper presents a new quality-control workflow, called MicroTalk, that requires some workers to Justify their reasoning and asks others to Reconsider their decisions after reading counter-arguments from workers with opposing views. Experiments on a challenging NLP annotation task with workers from Amazon Mechanical Turk show that (1) argumentation improves the accuracy of individual workers by 20%, (2) restricting consideration to workers with complex explanations improves accuracy even more, and (3) our complete MicroTalk aggregation workflow produces much higher accuracy than simpler voting approaches for a range of budgets.
DOI:
10.1609/hcomp.v4i1.13270
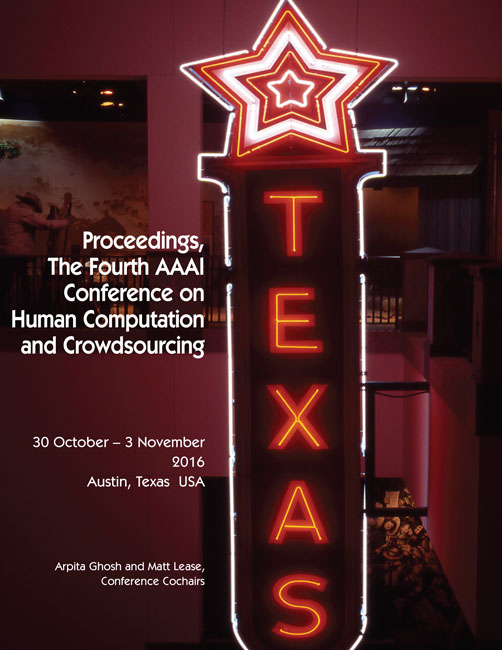
HCOMP
Vol. 4 (2016): Fourth AAAI Conference on Human Computation and Crowdsourcing
ISBN 978-1-57735-774-2