The 38th Annual AAAI Conference on Artificial Intelligence
February 20-27, 2024 | Vancouver, Canada
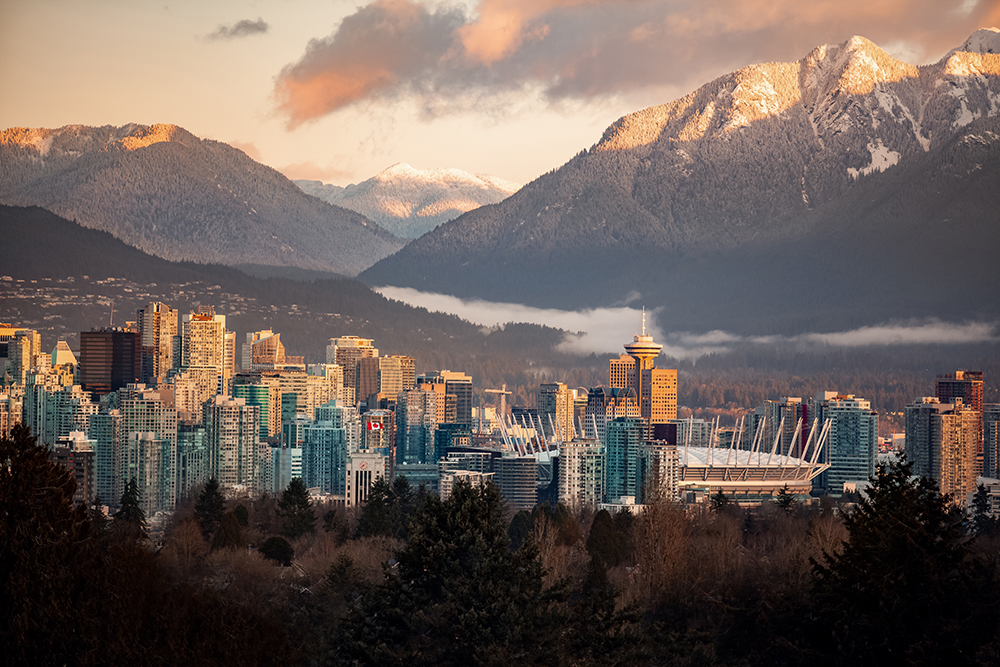
Twenty-Ninth AAAI/SIGAI Doctoral Consortium
Collocated with the Thirty-Eighth AAAI Conference on Artificial Intelligence
Sponsored by the Association for the Advancement of Artificial Intelligence
AAAI and ACM/SIGAI invite students to apply for the Twenty-Seventh AAAI/SIGAI Doctoral Consortium. The Doctoral Consortium (DC) provides an opportunity for a group of Ph.D. students to discuss and explore their research interests and career objectives with a panel of established researchers in artificial intelligence. The consortium has the following objectives: (1) to provide a setting for feedback on participants’ current research and guidance on future research directions; (2) to develop a supportive community of scholars and a spirit of collaborative research; (3) to support a new generation of diverse researchers by offering advice about academic, research, industry, and nontraditional career paths; and (4) to contribute to the overall conference goals, through interactions with other researchers and through participation in conference events.
The Doctoral Consortium will be held as a workshop on February 21, 2023 from 2-6pm, immediately before the start of the main AAAI conference. Student participants in the Doctoral Consortium will receive complimentary conference registration.
Program Overview: Room 210
Knowledge Distillation from Single-Task Teachers to Multi-Task Student for End-to-End Autonomous Driving
Pedram Agand
Does Robin Hood Use a Lightsaber?: Automated Planning for Storytelling
Nisha Simon
The Promise of Serverless Computing within Peer-to-Peer Architectures for Distributed ML Training
Amine Barrak
Identifying, Mitigating, and Anticipating Bias in Algorithmic Decisions
Joachim Baumann
Target Focused Shallow Transformer Framework for Efficient Visual Tracking
Md Maklachur Rahman
Towards Trustworthy Autonomous Systems via Conversations and Explanations
Balint Gyevnar
Temporal Dependencies and Spatio-Temporal Patterns of Time Series Models
Md. Khairul Islam
Autonomous Policy Explanations for Effective Human-Machine Teaming
Aaquib Tabrez
Data Efficient Paradigms for Personalized Assessment of Black-Box Taskable AI Systems
Pulkit Verma
AI-Assisted Human Teamwork
Sangwon Seo
Learning Pattern-Based Extractors from Natural Language and Knowledge Graphs: Applying Large Language Models to Wikipedia and Linked Open Data
Célian Ringwald
Neuro-Symbolic Integration for Reasoning and Learning on Knowledge Graphs
Luisa Werner
Risk Management in Image Generative Models through Model Fingerprinting
Changhoon Kim
Thesis Summary: Operationalizing User-Inclusive Transparency in Artificial Intelligence Systems
Deepa Muralidhar
Semi-factual Explanations in AI
Saugat Aryal
Visual Abstract Reasoning in Computational Imagery
Yuan Yang
Making AI Policies Transparent to Humans through Demonstrations
Michael S. Lee
Domain Engineering to Represent Human Behavior Using Multi-Agent Planning and Inductive Methodologies
Salena Torres Ashton
A Privacy Preserving Federated Learning (PPFL) Based Cognitive Digital Twin (CDT) Framework for Smart Cities
Sukanya Mandal
Learning Neuro-Symbolic Abstractions for Robot Planning and Learning
Naman Shah
The Inter-batch Diversity of Samples in Experience Replay for Continual Learning
Andrii Krutsylo
Deep Reinforcement Learning for Communication Networks
Raffaele Galliera
The Generalization and Robustness of Transformer-Based Language Models on Commonsense Reasoning
Ke Shen
Learning Generalizable and Composable Abstractions for Transfer in Reinforcement Learning
Rashmeet Kaur Nayyar
Learning from an Infant’s Visual Experience
Deepayan Sanyal
Discovering Heterogeneous Causal Effects in Relational Data
Shishir Adhikari
Tiered Coalition Formation Game Stability and Simulation
Nathan Arnold
Navigating Uncertainty in Epidemic Contexts with Reinforcement Learning
Elizabeth Akinyi Ondula
A Hybrid AI Framework for Sensor-Based Personal Health Monitoring towards Precision Health
Mbithe Nzomo
To Know the Causes of Things: Text Mining for Causal Relations
Fiona Anting Tan
For More Information
Inquiries concerning doctoral consortium submissions and suggestions may be directed to the doctoral consortium co-chairs at aaai24dcchairs@aaai.org. All other inquiries should be directed to AAAI at aaai24@aaai.org.
Doctoral Consortium Co-Chairs
Jakob Foerster (University of Oxford, UK)
Scott Sanner (U. of Toronto)