The 38th Annual AAAI Conference on Artificial Intelligence
February 20-27, 2024 | Vancouver, Canada
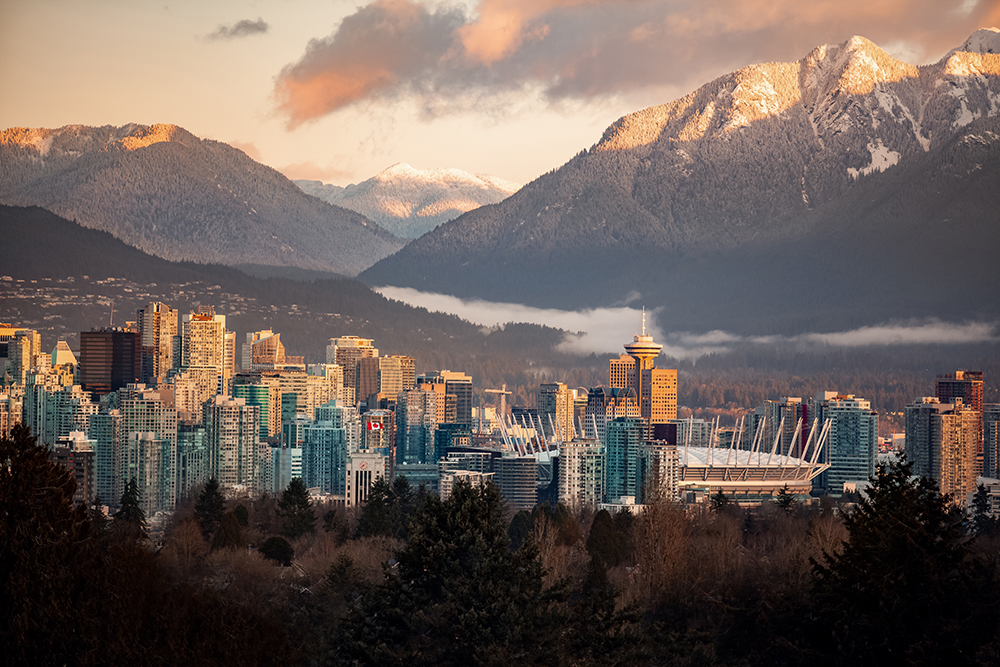
AAAI-24/IAAI-24/EAAI-24 Invited Speakers
Sponsored by the Association for the Advancement of Artificial Intelligence
February 22-25, 2024 | Vancouver Convention Centre – West Building | Vancouver, BC, Canada
Thursday, February 22
8:30AM – 9:25AM
Welcome and Opening Plenary Session
5:05PM – 7:00PM
AAAI Award for AI for the Benefit of Humanity
ML+Optimization: Driving Social Impact in public health and conservation, Milind Tambe
AAAI Invited Talk
Geometric ML: from Euclid to drug design, Michael Bronstein
Friday, February 23
8:30AM – 9:25AM
2024 Robert S. Engelmore Memorial Lecture Award
Accelerating AVs with the next generation of generative AI, Raquel Urtasun
9:30AM – 10:30AM
AAAI Invited Talk
Smart Advice: Intelligent Agents Assisting Humans in the Super AI Era, Sarit Kraus
5:05PM – 7:00PM
AAAI/IAAI Invited Talk
Objective-Driven AI: Towards Machines that Can Learn, Reason, and Plan Yann LeCun
AAAI Invited Talk
How Children Learn, Elizabeth S. Spelke
Saturday, February 24
8:30AM – 9:25AM
IAAI Invited Talk
Toward Foundational Robot Manipulation Skills, Dieter Fox
2:00PM – 3:00PM
AAAI/EAAI Patrick Henry Winston Outstanding Educator Award
AI Education in the Age of AI, Charles Isbell and Michael Littman
AAAI Classic Paper Award
Maximum Entropy Inverse Reinforcement Learning, Brian Ziebart, Andrew Maas, Andrew Bagnell, Anind Dey
3:45PM – 4:30PM
AAAI Organizational & Conference Awards
4:30PM – 6:00PM
Sunday, February 25
8:30AM – 9:25AM
AAAI Invited Talk
The Role of Rationality in Modern AI, Leslie Pack Kaelbling
5:05PM – 6:00PM
AAAI Invited Talk
Machines Make Up Stuff: Why Do Generative Models Hallucinate?, Pascale Fung
AAAI Award for AI for the Benefit Humanity
ML+Optimization: Driving Social Impact in public health and conservation
Milind Tambe, Harvard University/Google Research
For more than 15 years, my team and I have been focused on AI for social impact, deploying end-to-end systems in areas of public health, conservation and public safety. In this talk, I will highlight the results from our deployments for social impact in public health and conservation, as well as required innovations in integrating machine learning and optimization. Within public health, I will present recent results from our work in India with the world’s two largest mobile health programs for maternal and child care that have served millions of beneficiaries. Additionally, I will highlight results from an earlier project on HIV prevention among youth experiencing homelessness in Los Angeles. Turning to conservation, I will highlight efforts for protecting endangered wildlife in national parks around the globe. To address challenges of ML+optimization common to all of these applications, we have advanced the state of the art in decision-focused learning, restless multi-armed bandits, influence maximization in social networks and green security games. In pushing this research agenda, our ultimate goal is to empower local communities and non-profits to directly benefit from advances in AI tools and techniques.
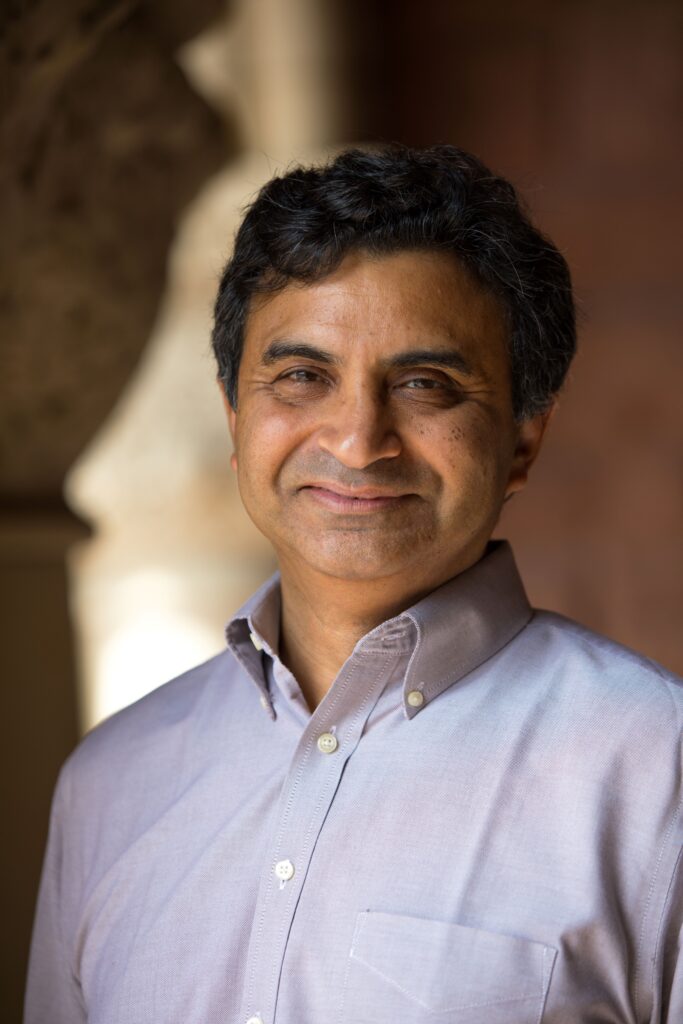
Milind Tambe is Gordon McKay Professor of Computer Science and Director of Center for Research in Computation and Society at Harvard University; concurrently, he is also Principal Scientist and Director for “AI for Social Good” at Google Research. He is recipient of the IJCAI John McCarthy Award, AAAI Feigenbaum Prize, AAAI Robert S. Engelmore Memorial Lecture Award, AAMAS ACM Autonomous Agents Research Award, INFORMS Wagner prize for excellence in Operations Research practice and MORS Rist Prize. He is a fellow of AAAI and ACM. For his work on AI and public safety, he has received Columbus Fellowship Foundation Homeland security award and commendations and certificates of appreciation from the US Coast Guard, the Federal Air Marshals Service and airport police at the city of Los Angeles.
AAAI Invited Talk
Geometric ML: from Euclid to drug design
Michael Bronstein, University of Oxford
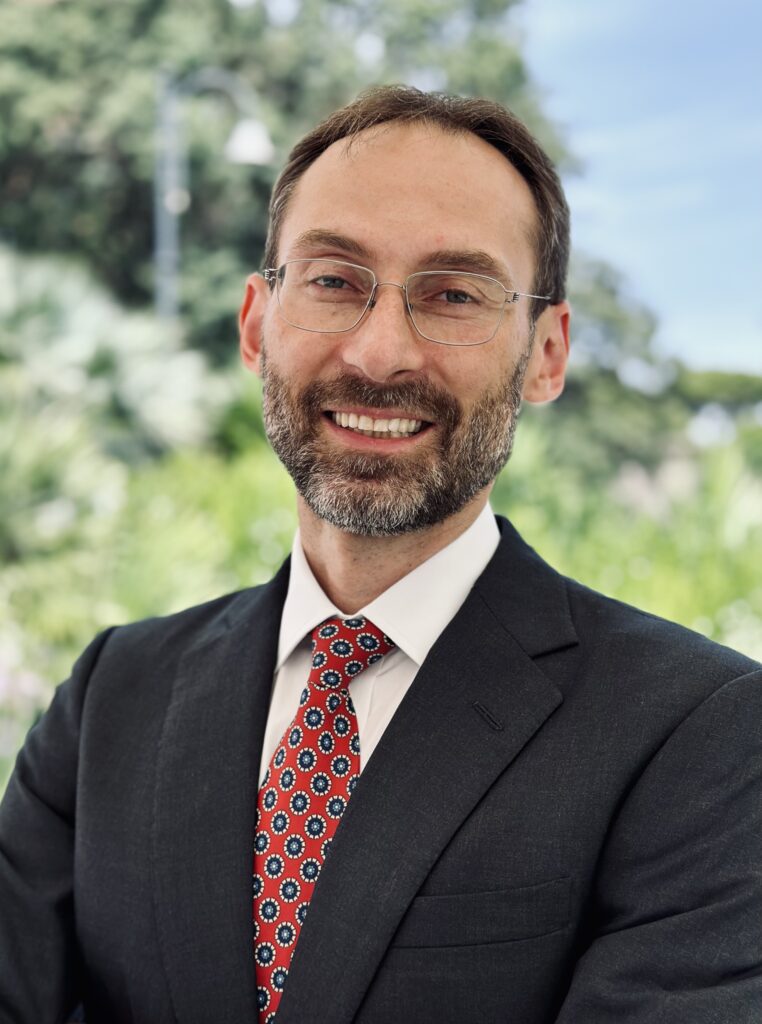
Michael Bronstein is the DeepMind Professor of AI at the University of Oxford. He previously served as Head of Graph Learning Research at Twitter, professor at Imperial College London, and held visiting appointments at Stanford, MIT, and Harvard. He is the recipient of the Royal Society Wolfson Research Merit Award, Royal Academy of Engineering Silver Medal, Turing World-Leading AI Research Fellowship, five ERC grants, two Google Faculty Research Awards, and two Amazon AWS ML Research Awards. He is a Member of the Academia Europaea, Fellow of IEEE, IAPR, BCS, and ELLIS, ACM Distinguished Speaker, and World Economic Forum Young Scientist. In addition to his academic career, Michael is a serial entrepreneur and founder of multiple startup companies, including Novafora, Invision (acquired by Intel in 2012), Videocites, and Fabula AI (acquired by Twitter in 2019).
IAAI Invited Talk
Toward Foundational Robot Manipulation Skills
Dieter Fox, NVIDIA
The last years have seen astonishing progress in the capabilities of generative AI techniques, particularly in the areas of language and visual understanding. Key to the success of these models is the availability of very large sets of images and text along with models that are able to digest such large datasets. Unfortunately, we have not been able to replicate this success in the context of robotics, where robots still struggle to perform seemingly simple tasks such as manipulating objects in the real world. A crucial reason for this problem is the lack of data suitable to train powerful, general models for robot decision making and control.
In this talk, I will describe our ongoing efforts toward developing the models and generating the kind of data that might enable us to train foundational robot manipulation skills. To generate large amounts of demonstration data, we sample many object rearrangement tasks in physically realistic simulation environments, generate high quality solutions for them, and then train perception-driven manipulation skills that can be used in unknown, real-world environments. We believe that such skills along with generative AI reasoning can provide robots with the capabilities necessary to succeed across a wide range of applications.
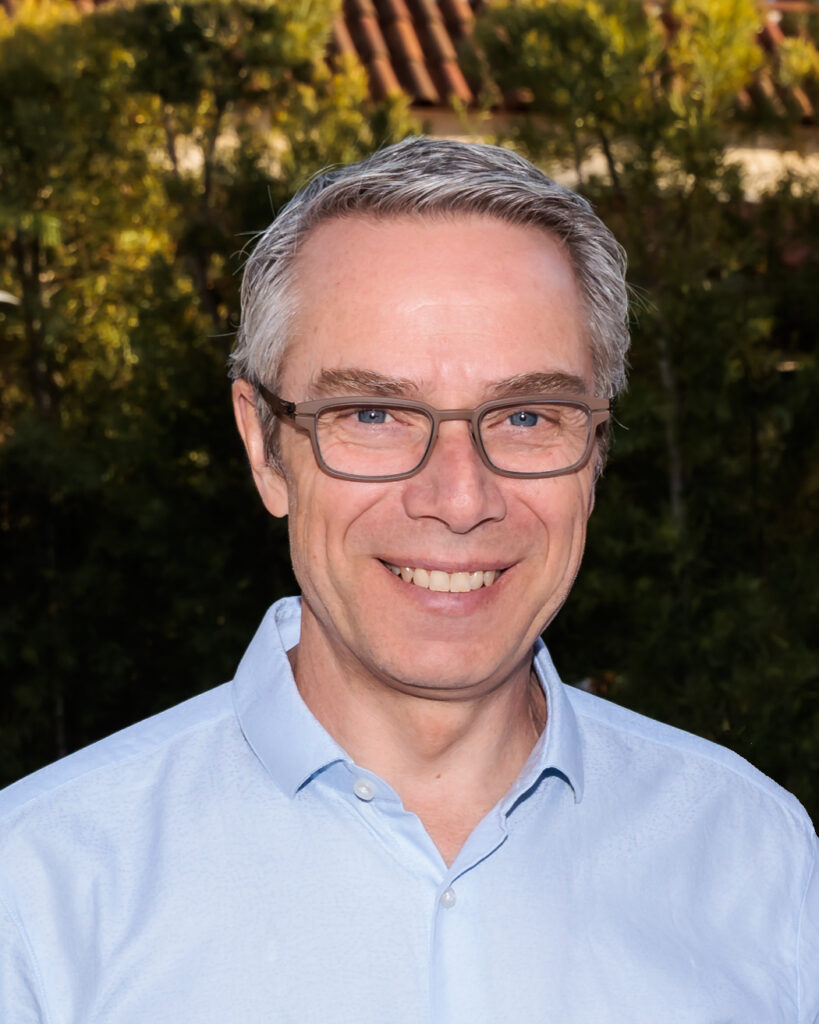
Dieter Fox is Senior Director of Robotics Research at NVIDIA and Professor in the Paul G. Allen School of Computer Science & Engineering at the University of Washington, where he heads the UW Robotics and State Estimation Lab. Dieter obtained his Ph.D. from the University of Bonn, Germany. His research is in robotics and artificial intelligence, with a focus on state estimation and perception applied to problems such as robot manipulation, mapping, and object detection and tracking. He has published more than 200 technical papers and is the co-author of the textbook “Probabilistic Robotics”. He is a Fellow of the IEEE, AAAI, and ACM, and recipient of the 2020 Pioneer in Robotics and Automation Award and the 2023 John McCarthy Award. Dieter also received several best paper awards at major robotics, AI, and computer vision conferences. He was an editor of the IEEE Transactions on Robotics, program co-chair of the 2008 AAAI Conference on Artificial Intelligence, and program chair of the 2013 Robotics: Science and Systems conference.
AAAI Invited Talk
Machines Make Up Stuff: Why Do Generative Models Hallucinate?
Pascale Fung, The Hong Kong University of Science & Technology
Generative AI models such as ChatGPT and Stable Diffusion have greatly accelerated the development and adoption of AI applications. However, one of the key challenges today is AI hallucination – generative models tend to confabulate plausible even authoritative sounding responses that are incorrect and non-factual, leading to downstream harm if not mitigated. Some findings showed that close to 30% of chatbot responses are hallucinations. Why do they hallucinate? And why popular methods such as RLHF and safety fine-tuning cannot completely mitigate this problem? In this talk, I will give an overview of the known causes of hallucination and methods to mitigate them, including in multimodal and multilingual scenarios. I will also talk about the remaining challenges and future directions in AI hallucination research.
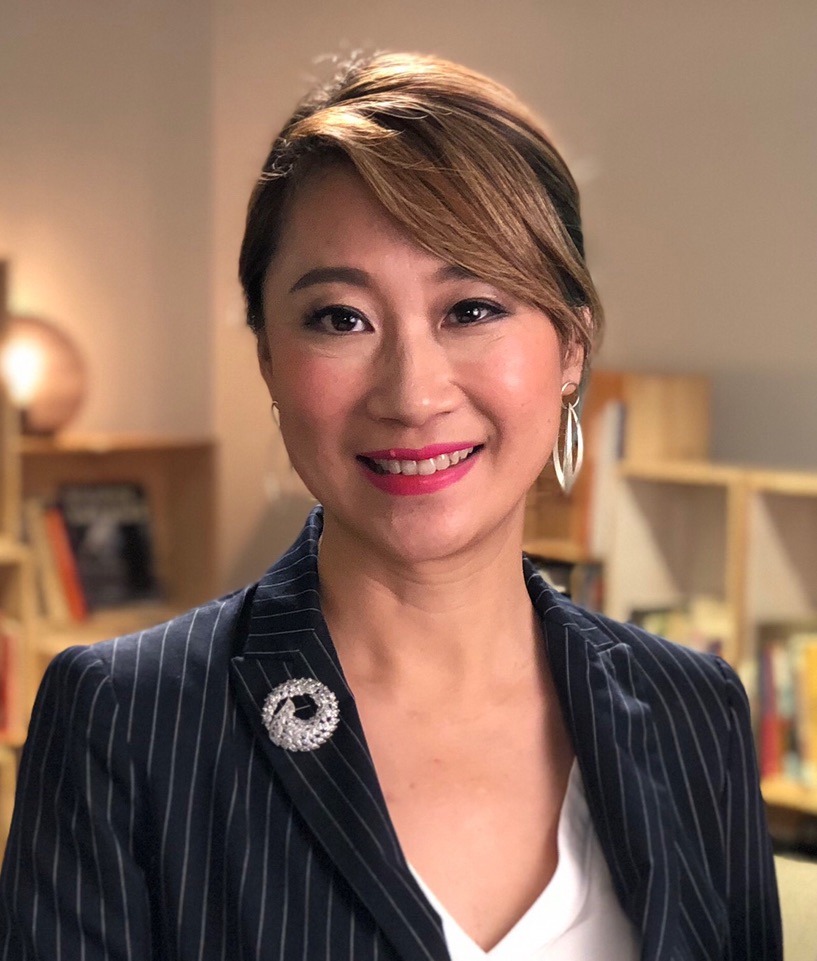
Pascale Fung is a Chair Professor at the Department of Electronic & Computer Engineering at The Hong Kong University of Science & Technology (HKUST), and a visiting professor at the Central Academy of Fine Arts in Beijing. She is the Director of HKUST Centre for AI Research (CAiRE) at HKUST. She is a Fellow of the AAAI, ACL, IEEE and ISCA. She is an expert on the Global Future Council for AI of the World Economic Forum. She represents HKUST on Partnership on AI to Benefit People and Society. She is on the Board of Governors of the IEEE Signal Processing Society. She is a member of the IEEE Working Group to develop an IEEE standard – Recommended Practice for Organizational Governance of Artificial Intelligence. She was the Distinguished Consultant on RAI at Meta in 2022 and a Faculty Visiting Researcher at Google in fall 2023. She served as Editor and Associate Editor for Computer Speech and Language, IEEE/ACM Transactions on Audio, Speech and Language Processing, Transactions for ACL, Journal of Machine Learning and others. Her team has won several best and outstanding paper awards at ACL, ACL and NeurIPS workshops. She is listed as one of the Forbes 50 over 50 Asia 2024.
AAAI/EAAI Patrick Henry Winston Outstanding Educator Award
AI Education in the Age of AI
Charles Isbell, University of Wisconsin-Madison and Michael L. Littman, Brown University
Charles and Michael are engaged in a decades-long conversation on AI, education, and AI education, exploring where it has been, where it could go, and why it is such a central part of so much that we all do. Occasionally that conversation has found its way into research and educational spaces, including two ongoing online classes in ML and RL taken by about 3300 students each year, a couple of music videos, and a series of educational videos on computing. They believe that this topic is so important and so serious that it must be addressed with a playful frame of mind—no one makes their best decisions and learns effectively when they think the well-being of the world is at stake. In this presentation, Charles and Michael continue their conversation with EAAI with the help of a moderator, answering questions about their approach to AI education and their outlook for the future. They promise to take it just as seriously as they do everything else… and probably there won’t be any singing.
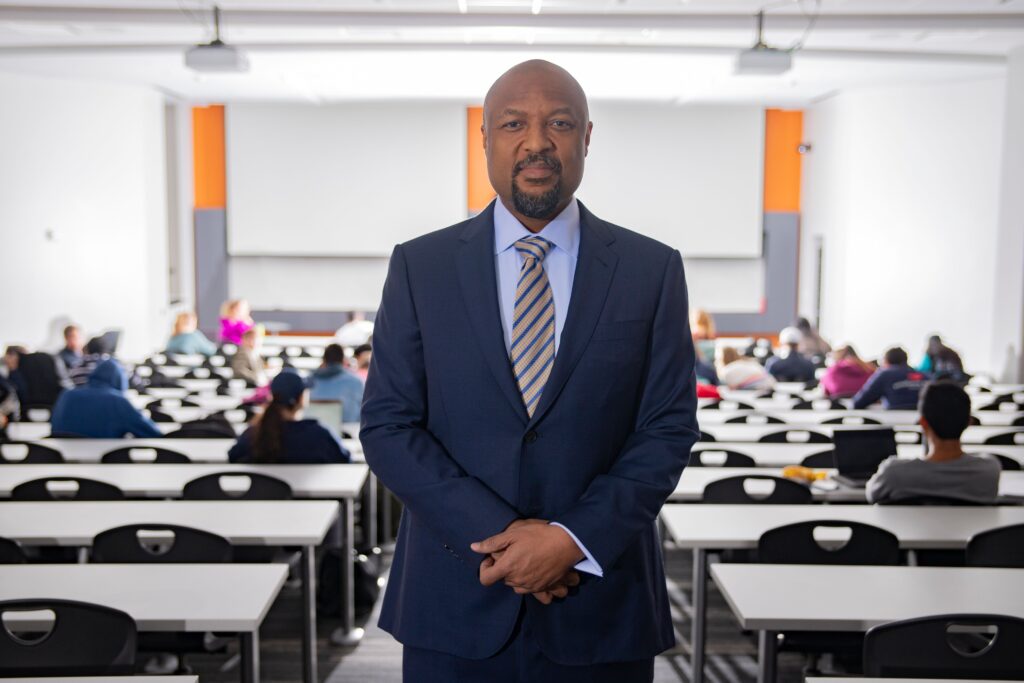
Charles Lee Isbell Jr. is an American computationalist, researcher, and educator. He is Provost and Vice Chancellor for Academic Affairs at the University of Wisconsin–Madison. Before joining the faculty there, he was a professor at the Georgia Institute of Technology College of Computing starting in 2002, and served as John P. Imlay, Jr. Dean of the College from July 2019 to July 2023. His research interests focus on machine learning and artificial intelligence, particularly interactive and human-centered AI. He has published over 100 scientific papers.[1] In addition to his research work, Isbell has been an advocate for increasing access to and diversity in higher education.
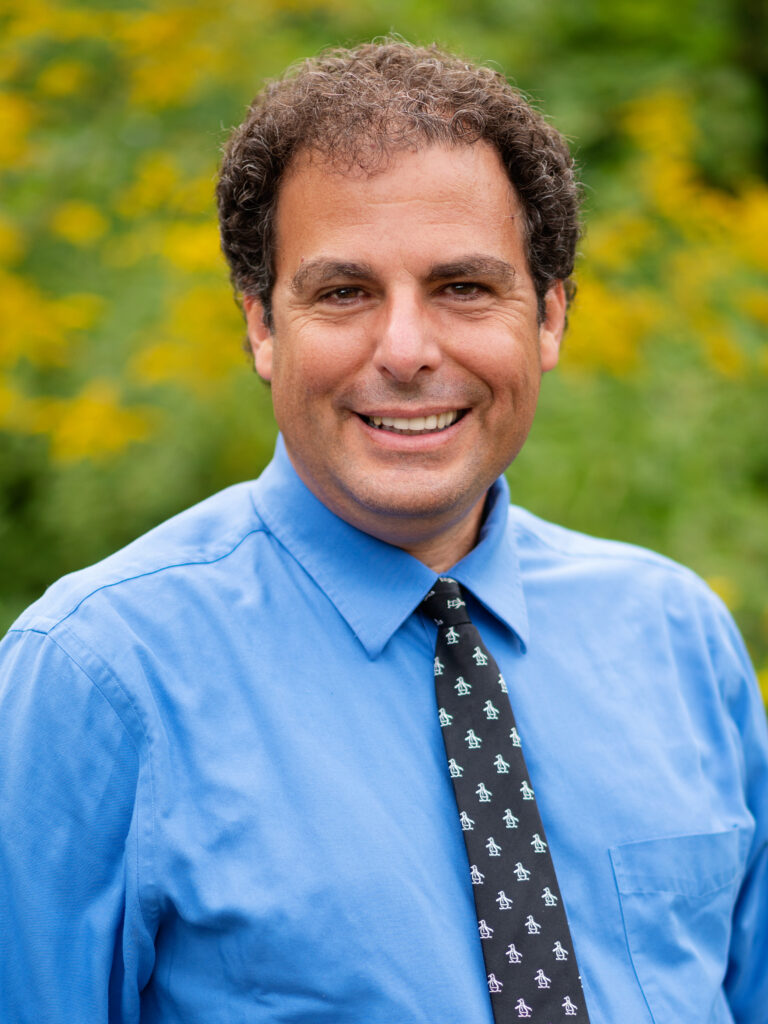
Michael L. Littman is University Professor of Computer Science at Brown University, where he studies machine learning and decision-making under uncertainty. He has earned multiple university-level awards for teaching and his research has been recognized with three best-paper awards and three influential paper awards. Littman is a Fellow of the Association for the Advancement of Artificial Intelligence and the Association for Computing Machinery. He is currently serving as Division Director for Information and Intelligent Systems at the National Science Foundation. His book “Code to Joy: Why Everyone Should Learn a Little Programming” (MIT Press) was released Fall 2023.
AAAI Invited Talk
The Role of Rationality in Modern AI
Leslie Pack Kaelbling, MIT
The classical approach to AI was to design systems that were rational at run-time: they had explicit representations of beliefs, goals, and plans and ran inference algorithms, online, to select actions. The rational approach was criticized (by the behaviorists) and modified (by the probabilists) but persisted in some form. Now the overwhelming success of the connectionist approach in so many areas presents evidence that the rational view may no longer have a role to play in AI. I will examine this question from several perspectives, including whether the rationality is present at design-time and/or at run-time, and whether systems with run-time rationality might be useful from the perspectives of computational efficiency, cognitive modeling and safety. I will present some current research focused on understanding the roles of learning in runtime-rational systems with the ultimate aim of constructing general-purpose human-level intelligent robots.
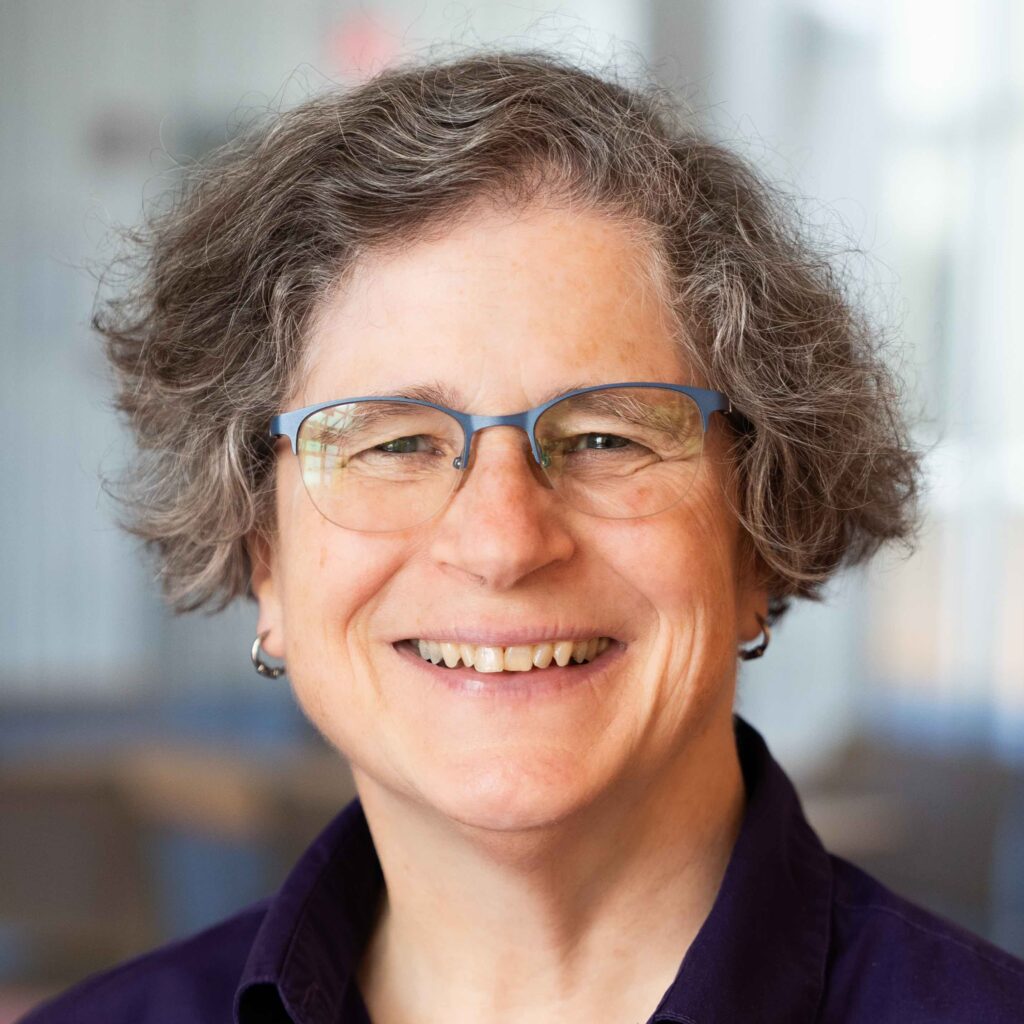
Leslie is a Professor at MIT. She has an undergraduate degree in Philosophy and a PhD in Computer Science from Stanford, and was previously on the faculty at Brown University. She was the founding editor-in-chief of the Journal of Machine Learning Research. Her research agenda is to make intelligent robots using methods including estimation, learning, planning, and reasoning. She is not a robot.
AAAI Invited Talk
Smart Advice: Intelligent Agents Assisting Humans in the Super AI Era
Sarit Kraus, Bar-Ilan University
The capabilities, autonomy, and efficiency of software and physical agents have increased remarkably in recent times. However, the rapid advancement of these intelligent systems presents significant challenges for humans when they need to interact, coordinate, or collaborate with them. Intelligent advising agents providing guidance to people in such situations can enhance their decision-making and alleviate cognitive load. Alongside identifying valuable advice, these assisting agents must also deliver explanations to convince humans that the provided advice is advantageous and trustworthy. In the presentation, we will highlight the challenges that emerge when humans need to collaborate with drones, autonomous vehicles, and intelligent systems for negotiation and mediation. Additionally, we will introduce several approaches for developing assisting agents aimed at enhancing human performance and satisfaction.
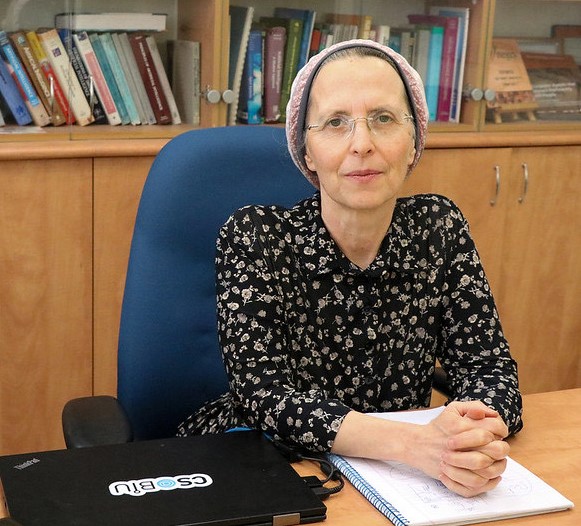
Sarit Kraus is a Professor of Computer Science at Bar-Ilan University. Her research is focused on intelligent agents and multi-agent systems integrating machine-learning techniques with optimization and game theory methods. In particular, she studies the development of intelligent agents that can interact proficiently with people and with robots.
For her work, she received many prestigious awards. She was awarded the IJCAI Computers and Thought Award, the IJCAI Research Excellent Award, the ACM SIGART Agents Research Award, the ACM Athena Lecturer, the EMET prize, and was twice the winner of the IFAAMAS influential paper award. She is an ACM, AAAI and EurAI fellow and a recipient of the advanced ERC grant. She is an elected member of the Israel Academy of Sciences and Humanities.
AAAI/IAAI Invited Talk
Objective-Driven AI: Towards Machines that can Learn, Reason, and Plan
Yann LeCun, Meta-FAIR & New York University
How could machines learn as efficiently as humans and animals? How could machines learn how the world works and acquire common sense? How could machines learn to reason and plan? Current AI architectures, such as Auto-Regressive Large Language Models fall short. I will propose a modular cognitive architecture that may constitute a path towards answering these questions. The centerpiece of the architecture is a predictive world model that allows the system to predict the consequences of its actions and to plan a sequence of actions that optimize a set of objectives. The objectives include guardrails that guarantee the system’s controllability and safety. The world model employs a Hierarchical Joint Embedding Predictive Architecture (H-JEPA) trained with self-supervised learning. The JEPA learns abstract representations of the percepts that are simultaneously maximally informative and maximally predictable. The corresponding working paper is available here: https://openreview.net/forum?id=BZ5a1r-kVsf
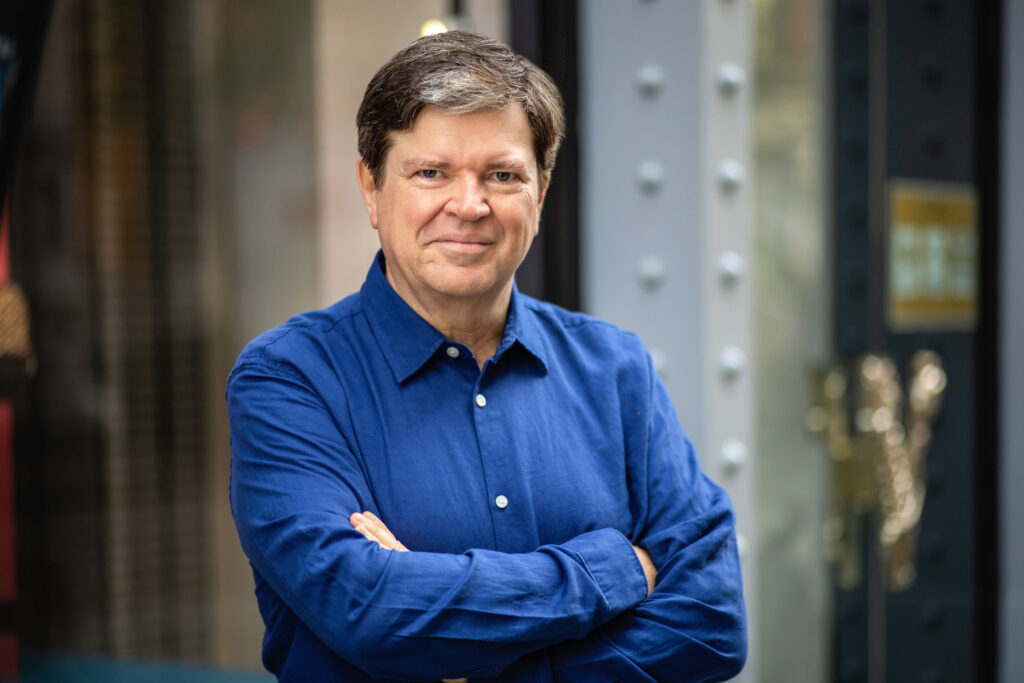
Yann LeCun is VP & Chief AI Scientist at Meta and Silver Professor at NYU affiliated with the Courant Institute of Mathematical Sciences & the Center for Data Science. He was the founding Director of FAIR and of the NYU Center for Data Science. He received an Engineering Diploma from ESIEE (Paris) and a PhD from Sorbonne Université. After a postdoc in Toronto he joined AT&T Bell Labs in 1988, and AT&T Labs in 1996 as Head of Image Processing Research. He joined NYU as a professor in 2003 and Meta/Facebook in 2013. His interests include AI, machine learning, computer perception, robotics, and computational neuroscience. He is the recipient of the 2018 ACM Turing Award (with Geoffrey Hinton and Yoshua Bengio) for “conceptual and engineering breakthroughs that have made deep neural networks a critical component of computing”, a member of the National Academy of Sciences, the National Academy of Engineering, the French Académie des Sciences. http://yann.lecun.com
AAAI Invited Talk
How Children Learn
Elizabeth S. Spelke, Harvard University
In their first years and with no instruction, children learn one or more natural languages; they develop a host of skills for moving themselves and manipulating objects; they learn the paths connecting places in their homes, the bonds connecting people in their social networks, and the forms and functions of everyday objects; and they deploy concepts of number, geometry, and causality that continue to guide the reasoning of adults, including mathematicians. How children do this is a great, unanswered question, but research on human infants, children, adults, and non-human animals, using diverse methods from the cognitive, brain, and computational sciences, provides some hints. It reveals at least seven domain-specific cognitive systems that emerge and function in infancy: six systems of core knowledge that are shared with other animals and represent places, objects, animate beings, social beings, number and geometry, and a seventh system that likely is unique to humans and underlies language learning during the first year. These automatic, unconscious, fixed systems function throughout life and provide inputs to the malleable, conscious perceptions and thoughts that power our endlessly inventive human minds.
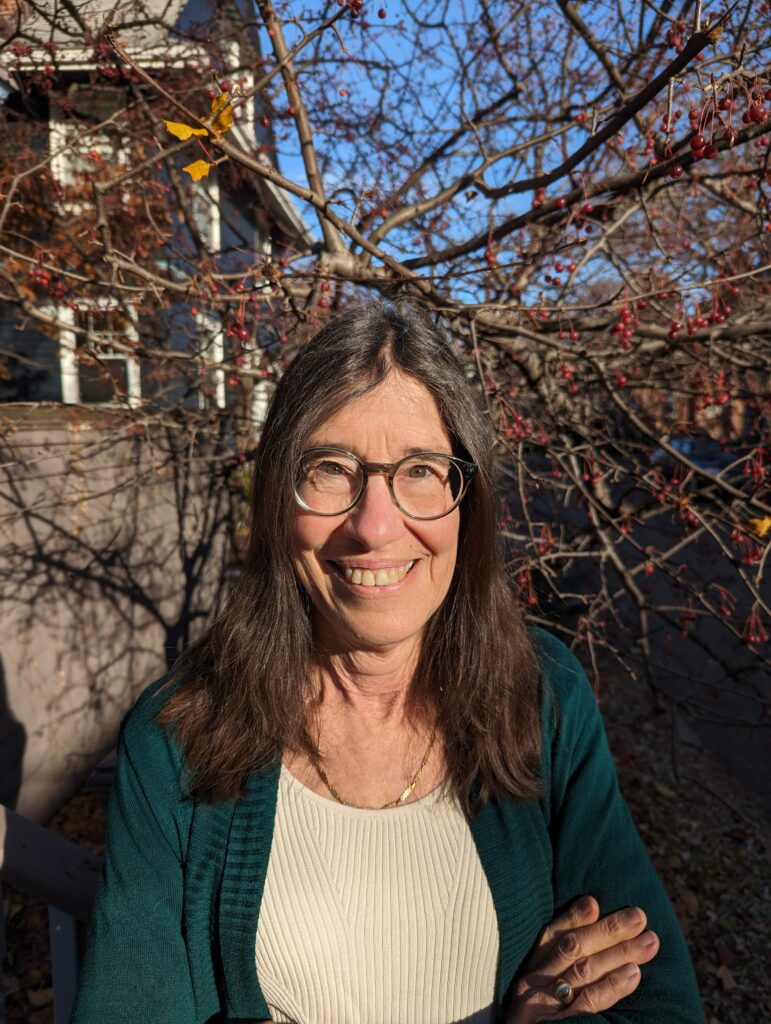
Elizabeth Spelke is the Marshall L. Berkman Professor of Psychology and an investigator at Center for Brains, Minds and Machines in Cambridge, MA. She studies the innate cognitive capacities that emerge in human infancy, summarized in her book, What Babies Know (2022), as well as children’s capacities for fast and flexible learning. With cognitive and comparative neuroscientists, she investigates whether the cognitive mechanisms driving young children’s learning are shared by other animals and continue to function in older children and adults. With computational cognitive scientists, she probes the mechanisms that give rise to intelligence. With economists, she leverages findings from the developmental cognitive sciences to create and evaluate interventions to enhance the learning of children worldwide, and she uses findings from field research evaluating the interventions to deepen understanding of how humans learn.
2024 Robert S. Engelmore Memorial Lecture Award
Accelerating AVs with the next generation of generative AI
Raquel Urtasun, Waabi
Despite meaningful progress over the past few decades, advances in the self-driving industry have plateaued. Traditional approaches are incredibly labor- and capital-intensive, relying on hand-engineered systems and millions of real-world testing miles. Generative AI offers the much-needed new path to unlocking autonomous vehicles at scale and changing the way these systems work.
In this lecture, Waabi CEO and Founder Raquel Urtasun will discuss how leveraging generative AI will evolve the way autonomous driving systems are developed and trained, resulting in faster, safer, and more scalable deployment of transformative self-driving technology worldwide.
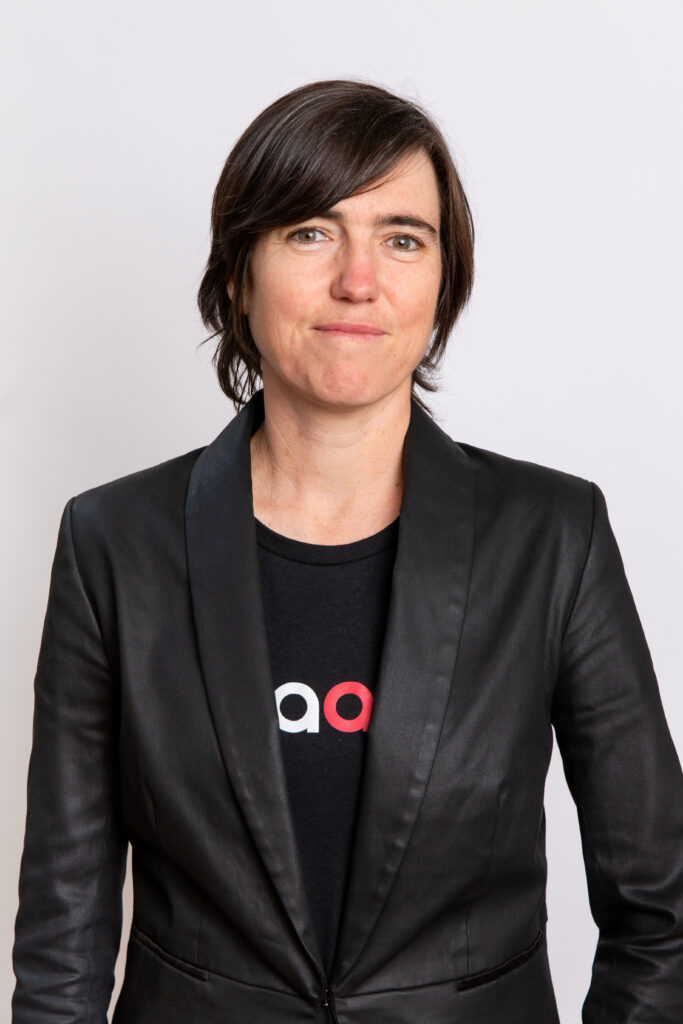
Raquel Urtasun is the Founder and CEO of Waabi, an AI company building the next generation of self-driving technology. Waabi is the culmination of Raquel’s 20-year career in AI and 10 years of experience building self-driving solutions. Raquel is also a Full Professor in the Department of Computer Science at the University of Toronto, a co-founder of the Vector Institute for AI, and the recipient of several high-profile awards including a Longuet-Higgins Prize, an Everingham Prize, an NSERC EWR Steacie Award, two NVIDIA Pioneers of AI Awards, three Google Faculty Research Awards, an Amazon Faculty Research Award, two Best Paper Runner up Prize awards at CVPR in 2013 and 2017 and more.