The 38th Annual AAAI Conference on Artificial Intelligence
February 20-27, 2024 | Vancouver, Canada
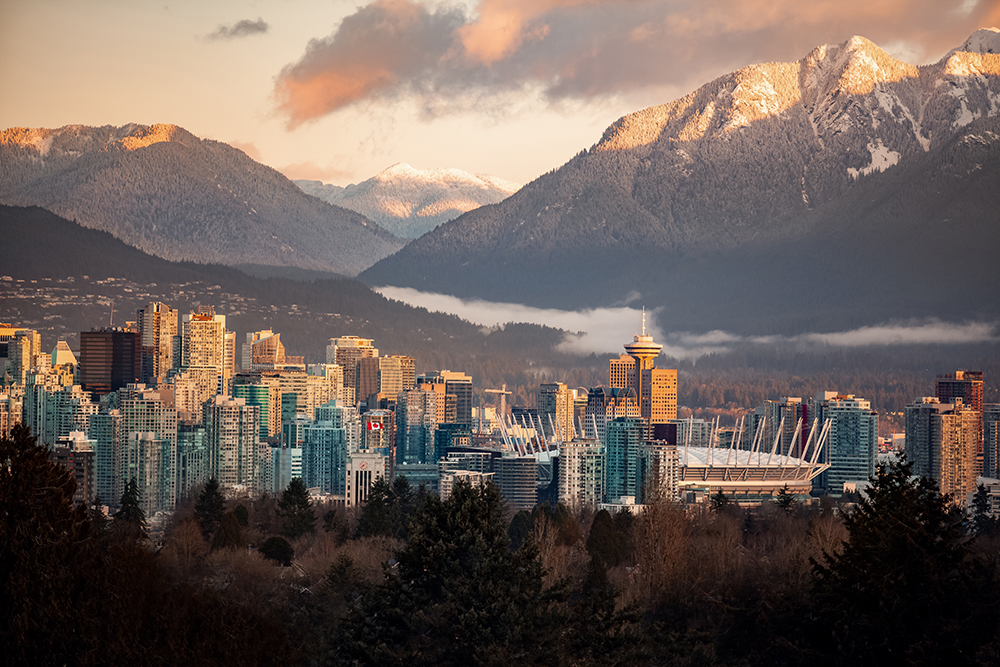
AAAI-24 Bridge Program
Sponsored by the Association for the Advancement of Artificial Intelligence
February 20-21, 2024 | Vancouver Convention Centre – West Building | Vancouver, BC, Canada
Cancelled – B7: Deep Automated Program and Proof Synthesis
B8: Exploring the use of Federated Learning for Data-Sensitive applications
B9: Knowledge-guided Machine Learning: Bridging Scientific Knowledge and AI
B1: AI for Financial Services
The goal of this bridge is to bring together AI researchers and practitioners from industry, government and academia, to share technical advances and insights of the application of AI techniques to financial services. The target audience is AI researchers that are actively working on the use of AI in financial institutions as well as researchers that would like to explore the potential application of their work to this domain.
For further and up to date information please check the Bridge website:
https://sites.google.com/view/aaai24-ai-fin
Format
The bridge will include invited talks, panels and tutorials covering a wide range of topics at the intersection of AI and Financial Services. The bridge will also include networking sessions and mentoring opportunities for students.
Submissions
The bridge would like to receive different types of submissions:
- Tutorials or System Demonstrations that introduce the main open problems and their potential solutions within AI / financial applications. Send proposals of tutorials to alberto.pozancolancho@jpmorgan.com. They should include:
- Topic of tutorial / System Demonstration
- Description of its contents
- Names and short bios of presenters
- Length (up to two hours)
- Extended abstracts of 2-4 pages in AAAI format that showcase the current application of AI in financial services. Extended abstracts will be reviewed using a double blind process. Please, remove any reference to authors in the paper. The page limits apply to the inclusion of any appendices or supplementary material to the paper which should be submitted in the same PDF. We encourage students to submit their current work. Selected applications will be asked to present a poster during the bridge, and might receive mentorship sessions from academia and industry experts.
Link to submissions: https://easychair.org/conferences/?conf=aifinbridge2024
Important Dates
- Submissions due: December 9th 2023
- Notification to authors: December 18th
- AAAI early registration deadline: December 20th
- Bridge at AAAI: February 20th
Organizers
Parisa Zehtabi, Ph.D.,
Vice President, J.P.Morgan AI Research, parisa.zehtabi@jpmorgan.com
Parisa Zehtabi is a research lead in JP Morgan mainly focusing on applications of AI planning and optimization in the financial sector. Before joining AI Research at JP Morgan, she was doing her PhD in Planning for Hybrid domains via Satisfiability Modula Theories (SMT) at King’s College London. Her research has been motivated by applying different planning techniques in real-world applications particularly robust planning for hybrid domains. She is regularly involved as PC member in ICAPS, and has organized the last two editions of the Workshop on Planning and Scheduling for Financial Services (FinPlan) at ICAPS.
Alberto Pozanco, Ph.D.,
Vice President, J.P.Morgan AI Research, alberto.pozancolancho@jpmorgan.com
Alberto Pozanco is a research lead at JP Morgan AI Research, where he joined after receiving his PhD in Computer Science from Universidad Carlos III de Madrid. The main focus of his current work includes the use of automated planning and optimization techniques to solve real world problems at JP Morgan. His research interests extend to other related Artificial Intelligence areas such as reinforcement learning, heuristic search and knowledge representation. He is regularly involved as PC member in conferences such as AAAI, IJCAI or ICAPS, and has organized the last two editions of the Workshop on Planning and Scheduling for Financial Services (FinPlan) at ICAPS.
Stefan Zohren, Ph.D.,
Associate Professor, Oxford University, stefan.zohren@eng.ox.ac.uk
Stefan Zohren is an Associate Professor at Engineering Science, Man Group Research Fellow in Financial Machine Learning and former Deputy Director of the Oxford-Man Institute of Quantitative Finance, a Research Associate at the Oxford Internet Institute and a Mentor in the FinTech stream at the Creative Destruction Lab at Said Business School, all at the University of Oxford. He also works on commercial projects with Man Group, the funding partner of the Oxford-Man Institute, firstly as a Scientific Advisor and later as Principle Quant. Stefan is regularly involved in organising workshops at venues such as SIAM conference on math finance or ICAIF where he is also part of the senior program committee.
Ramin Hassani, Ph.D.,
AI Scientist at MIT CSAIL,
rhasani@csail.mit.edu
Ramin Hasani is an AI Scientist at the Computer Science and Artificial Intelligence Lab (CSAIL), Massachusetts Institute of Technology (MIT). Previously, he was jointly appointed as a Principal AI and Machine Learning Scientist at the Vanguard Group and a Research Affiliate at CSAIL MIT. Ramin’s research focuses on robust deep learning and decision-making in complex dynamical systems. Prior to that, he was a Postdoctoral Associate at CSAIL MIT, leading research on modeling intelligence and sequential decision making, with Prof. Daniela Rus. He received his Ph.D. degree with distinction in Computer Science from the Vienna University of Technology (TU Wien), Austria (May 2020). His Ph.D. dissertation and continued research on Liquid Neural Networks got recognized internationally with numerous nominations and awards such as TU¨ V Austria Dissertation Award nomination in 2020, and HPC Innovation Excellence Award in 2022. He is a frequent TEDx Speaker.
Guiling Wang, Ph.D.,
Distinguished Professor and Associate Dean for Research, Ying Wu College of Computing,
gwang@njit.edu
Grace Wang is currently a distinguished professor and the associate dean for research of YingWu College of Computing. She also holds a joint appointment at the Martin Tuchman School of Management and the Data Science Department. She received her Ph.D. in Computer Science and Engineering and a minor in Statistics from The Pennsylvania State University in 2006. Her research interests include Fin-Tech, applied deep learning, blockchain technologies, and intelligent transportation.
Daniel Borrajo, Ph.D.,
Executive Director, J.P. Morgan,AI Research,
daniel.borrajo@jpmorgan.com
Daniel Borrajo is a Research Director at J.P. Morgan AI Research. He is also a Professor at Universidad Carlos III de Madrid (on leave), where he was Head of the Computer Science Department, Vicedean of Computer Science Degree and Head of the planning and Learning Group. He has more than 35 years of experience of work on AI, from the research side as well as developing AI solutions for companies. His main research interests are in the integration of the two main AI paradigms: model-based (e.g. AI Planning) and modelfree (e.g. Machine learning). He has been Program Chair of AI-related international conferences, regularly serves in the program committee of leading international AI conferences, and he is currently Associate Editor of the Artificial Intelligence Journal.
Nazanin Mehrasa, Ph.D.,
Senior Machine Learning Researcher at Borealis AI, nazanin.mehrasa@borealisai.com
Nazanin Mehrasa is a Senior Machine Learning Researcher at Borealis AI, focusing on AI for financial services. She received her Ph.D. in computer science from Simon Fraser University in 2021. Her Ph.D. research primarily centered on event analysis in time-series data, where she studied probabilistic generative models for time-series, and learning algorithms in the context of partially labeled data.
Eric Jiawei He, Ph.D.,
Senior Machine Learning Research Team Lead at Borealis AI, jiawei.he@borealisai.com
Eric Jiawei is a Machine Learning Research Team Lead at Borealis AI focusing on AI in financial applications. He received his Ph.D. from Simon Fraser University in 2019. In 2019, he served as local chair of the Symposium on Advances in Approximate Bayesian Inference (AABI) and successfully organized the event in Vancouver that year. In 2021, Dr. He together with Dr. Seiradaki founded the Let’s SOLVE it undergraduate mentorship program, aiming to support under-represented groups in AI. In 2023, Dr. He lead organized the diversity and inclusion social event at CVPR 2023.
B2: AI for Materials Science
The development of new materials and production processes and the customization of existing ones is increasingly driven by AI, in particular Bayesian optimization and surrogate modeling. In many cases, materials science has relied on compute-intensive simulations to evaluate the properties of proposed designs, or the effect a change might have. Such simulations do not scale to the vast design spaces that materials scientists explore. Machine learning provides an alternative: properties are approximated through the predictions of surrogate models rather than computed by simulations, orders of magnitude faster.
Both AI and materials science are working on conceptually similar problems — how to efficiently identify the best design choices, be that for a machine learning pipeline or a new material. Yet, there is little collaboration between the communities. The purpose of this Bridge is to bring the communities closer together, facilitate cross-disciplinary collaborations, identify common problems, and develop plans for tackling them.
Submissions:
We solicit poster submissions that present novel applications, novel algorithms, or pose challenges at the intersection of AI and materials science, in the widest sense. Whether it’s a mature system or only an idea, we welcome your submissions. Areas of interest include, but are not limited to: Bayesian optimization, reinforcement learning, surrogate modeling, neural network approaches and their applications to design new materials and production processes, optimize existing materials and production processes, characterize or test materials, and monitor the performance of materials. Please feel free to contact the organizers informally for any questions.
Posters will undergo a light review by the organizers for suitability for the Bridge. Submissions are due November 18, notifications will be sent out December 2. Please submit a PDF version of your poster on Easychair at https://easychair.org/conferences/?conf=aimat24.
For more information, see the bridge website at https://sites.google.com/view/aimat24/home.
Organizers:
- Peter Collins, Iowa State University, pcollins@iastate.edu
- Peter Frazier, Cornell University, pf98@cornell.edu
- Roman Garnett, Washington University at St Louis, garnett@wustl.edu
- Patrick Johnson, Iowa State University, paj3@istate.edu
- Jessica Koehne, NASA, jessica.e.koehne@nasa.gov
- Lars Kotthoff, University of Wyoming, larsko@uwyo.edu
Peter Collins,
Iowa State University, pcollins@iastate.edu
Peter Collins is a Professor in the Department of Materials Science and Engineering at Iowa State University. His experiences and interests involve the practical and theoretical treatments of microstructure-property relationship, novel metal matrix composites, additive manufacturing techniques, and combinatorial materials science.
Peter Frazier,
Cornell University,
pf98@cornell.edu
Peter Frazier is a Professor in Operations Research and Information Engineering at Cornell University. He has made contributions to Bayesian optimization and to the application of AI in, among other areas, materials science, biochemistry, and medicine.
Roman Garnett,
Washington University at St Louis, garnett@wustl.edu
Roman Garnett is an Associate Professor in the Department of Computer Science and Engineering at Washington University in St. Louis. He has pioneered applications of AI for scientific discovery in many fields and is an expert on Bayesian optimization.
Patrick Johnson,
Iowa State University,
paj3@istate.edu
Patrick Johnson is a Professor in the Department of Materials Science and Engineering at Iowa State University. He has decades of experience developing nanomaterials, biomedical devices, and applications of machine learning and AI to the development of next-generation materials and devices.
Jessica Koehne,
NASA,
jessica.e.koehne@nasa.gov
Jessica Koehne is a research scientist at NASA Ames Research Center. She has spent the past 20 years developing a carbon nanofiber, carbon nanotube, and graphene-based sensor platforms for detection of DNA, rRNA, proteins and neurotransmitters, with applications ranging from point-of-care for astronaut health monitoring including implantable and wearable sensors to the detection of life signatures for planetary exploration.
Lars Kotthoff,
University of Wyoming, larsko@uwyo.edu
Lars Kotthoff is an Associate Professor in the Department of Electrical Engineering and Computer Science at the University of Wyoming. He has made foundational contributions to the field of automated machine learning and closely collaborates with engineering departments to apply AI in different areas, including materials science.
B3: Artificial Intelligence for Design Problems
We propose a bridge program on the role of Artificial Intelligence (AI) in various design tasks. AI-based tools can be beneficial for numerous aspects of design such as increasing automation, improving the efficiency of the design process, increasing the diversity of the designs, augmenting creativity, providing non-intuitive insights, and generating personalized feedback. Besides AI researchers, this interdisciplinary program brings together researchers from various fields such as physical design experts focusing on 2D and 3D engineering design; cyber-physical design experts focusing on aircraft and underwater vehicle design; and architects focusing on building design.
Recently, generative AI has been used for several design areas such as graphic design, fashion design, interior design, and furniture design. However, in various cases, a quantitative evaluation is missing. We focus on both qualitative and qualitative evaluation of designs based on domain-specific evaluation criteria. While there are multiple important aspects of the design process, in this program we consider the following aspects: 1) performance, 2) efficiency, and 3) diversity. We also consider diverse design tools including computer-aided design (CAD) tools and AR/VR mediums. We note that while AI offers numerous advantages, it should be used thoughtfully and in conjunction with human creativity and expertise. AI can assist and augment the design process, but the goal is not to replace the critical thinking and creativity that designers bring to their work.
Topics:
AI for cyber-physical design, AI for structural design, Human aspects of AI-in-the-loop design, AI for architectural design, Role of foundation models in AI for design.
Objectives:
The objective is to bring AI and design communities to grow awareness about the applications of AI is numerous design tasks.
Format of Bridge:
- Morning: Kick-off and overview (15 mins)
- Invited talks (30 mins each)
- Aspects of cyber-physical design and the role of AI.
- AI for structural design.
- Human aspects of AI-in-the-loop design.
- AI for architectural design.
- Role of foundation models in AI for design.
- Break/Lunch (1 hour)
- Panel discussion (1 hour)
- Poster session (2 hours)
- Break (30 mins)
- Demos (15 mins each)
- Aircraft design
- Underwater vehicle design
- Building design
- Concluding remarks and dissemination
Attendance:
20 people
Submission requirements:
4 pages without the references
Bridge Committee:
- Anirban Roy, SRI International
- Adam Cobb, SRI International
- Susmit Jha, SRI International
- Eric Yeh, SRI International
- Karthik Ramani, Purdue University
- Christopher McComb, Carnegie Mellon University
- Takuma Nakabayashi, Obayashi Corporation
Bridge External URL:
https://nusci.csl.sri.com/AAAI_AI_for_design
B4: Collaborative AI and modeling of humans
Advances in Artificial Intelligence (AI) methods have allowed them to surpass human performance on many well-defined tasks. However, most real-world problems, especially those involving humans, are hard to specify a priori. A principled way to address this is to allow AI systems to collaborate with humans, and thereby actively anticipate and adapt to humans’ needs and abilities. To enable such reasoning, AI must be equipped with computational models of human behavior. Such models have been heavily investigated in cognitive science and AI-adjacent fields such as human-AI interaction, human-computer interaction (HCI), and behavioral game theory. However, due to differences in research goals and experimental settings, these communities have operated more or less independently, with limited exchange of theories and methods. In this bridge program, we aim to bring together members of the communities relevant to human-AI collaboration and user modeling to exchange theories, perspectives, and methods
Topics of Interest
The space of disciplines covered by the relevant fields is very large and submissions are expected to cover topics such as
- Machine learning with human(s) in the loop
- User modeling, theory of mind, and computational rationality
- Human-AI collaboration
Format
This event will be one day long. It will start with two keynote talks, from the perspectives on either side of the bridge topic of human modeling in AI. Next, a tutorial will provide a deep dive into the bridge topic. This will be followed by a poster session where authors of accepted papers will be invited to present their work. The day will conclude with an interactive discussion with a panel of experts with ample time to discuss.
Submissions
We encourage submissions for the poster session on all topics relevant to the bridge but expect they include a dedicated section elucidating the potential interconnection of both disciplines. Submissions are reviewed double-blind, so they should be anonymized. There will be no proceedings, so papers that have been or will be submitted or published in other conferences or journals are also welcome.
We accept papers of 2 to 8 pages, excluding references and appendices. The papers should be formatted in the AAAI two-column, camera-ready style (see https://aaai.org/authorkit24-2 for details) and authors can submit their works through https://openreview.net/group?id=AAAI.org/2024/Workshop/CAIHu .
For more detailed submission instructions, please visit https://sites.google.com/view/collab-ai-and-human-modeling/call-for-papers.
Target Audience
In this bridge program, we hope to bring together a broad audience of students, researchers, and practitioners in fields relevant to human-AI collaboration and human behavior modeling including AI, HCI, and CogSci.
Organizers
- Andrew Howes; University of Exeter, England; A.Howes2@exeter.ac.uk
- Samuel Kaski; Aalto University, Finland and University of Manchester, UK; samuel.kaski@aalto.fi
- Frans A. Oliehoek; Delft University of Technology, Netherlands; f.a.oliehoek@tudelft
- Nuria Oliver; ELLIS Alicante, Spain; nuria@ellisalicante.org
- Matthew E. Taylor; University of Alberta & Alberta Machine Intelligence Institute, Canada; matthew.e.taylor@ualberta.ca
Andrew Howes;
University of Exeter, England;
A.Howes2@exeter.ac.uk
Professor Andrew Howes’ career started at the University of Lancaster where he was trained in Computer Science and where his Ph.D. supervisor, Professor Stephen J. Payne (1986-1989), was based. Afterwards, he then moved to the MRC Applied Psychology Unit in Cambridge and to Carnegie Mellon University in Pittsburgh where he conducted post-doctoral research on cognitive architectures under the supervision of Professor Richard M. Young (1989-1994).
He subsequently held posts at Cardiff University (Psychology) and University of Manchester (Informatics and Business), and Birmingham. He has also made sabbatical visits to NASA Ames Research Centre and during the winter of 2016, Professor Howes was the inaugural Marshall Weinberg Visiting Professor in the Department of Psychology at University of Michigan. He is now at the University of Exeter as the Head of Department of Computer Science in the Faculty of Environment, Science and Economy
Samuel Kaski;
Aalto University, Finland and University of Manchester, UK;
samuel.kaski@aalto.fi
Samuel Kaski is a professor of Computer Science at Aalto University and professor of AI in The University of Manchester. He leads the Finnish Center for Artificial Intelligence FCAI, ELLIS Unit Helsinki and the ELISE EU Network of AI Excellence Centres. He received the Turing AI World-Leading Researcher Fellowship in 2021. His field is probabilistic machine learning, with applications in new kinds of collaborative AI-assistants able to work well with humans in modeling, design and decision tasks. Application domains include computational biology and medicine, brain signal analysis, information retrieval and user modeling. Prof. Kaski is an ELLIS Fellow, UKRI Turing AI Fellow, and Turing Fellow of the Alan Turing Institute.
Frans A. Oliehoek;
Delft University of Technology, Netherlands;
f.a.oliehoek@tudelft
Dr. Frans A. Oliehoek is Associate Professor at Delft University of Technology, where he is a leader of the sequential decision making group, a scientific director of the Mercury machine learning lab, and director and co-founder of the ELLIS Unit Delft. He received his Ph.D. in Computer Science (2010) from the University of Amsterdam (UvA), and held positions at various universities including MIT, Maastricht University and the University of Liverpool. Frans’ research interests revolve around intelligent systems that learn about their environment via interaction, building on techniques from machine learning, AI and game theory. He has served as PC/SPC/AC at top-tier venues in AI and machine learning, and currently serves as associate editor for JAIR and AIJ. He is a Senior Member of AAAI, and was awarded a number of personal research grants, including a prestigious ERC Starting Grant.
Nuria Oliver;
ELLIS Alicante, Spain;
nuria@ellisalicante.org
Nuria Oliver is Director and one of the founders of the ELLIS Alicante Foundation. She is co-founder and vice-president of ELLIS.
During the COVID-19 pandemic, she was Commissioner to the President of the Valencian Government on AI and Data Science against COVID-19. She advises several universities, governments and companies. Previously, she was Director of Data Science Research at Vodafone, Scientific Director at Telefónica and researcher at Microsoft Research. She holds a PhD from the Media Lab at MIT and an Honorary Doctorate from the University Miguel Hernández. She is an IEEE Fellow, and ACM Fellow, and EurAI Fellow and elected permanent member of the Royal Academy of Engineering of Spain. She is also a member of CHI Academy and the Academia Europaea. She holds an Honorary Doctorate from the University Miguel Hernandez. She is well known for her work in computational models of human behavior, human computer-interaction, mobile computing and big data for social good. Named inventor of 40 patents. She has received many awards, including the MIT TR100 Young Innovator Award (2004), Spanish National Computer Science Award (2016), Engineer of the Year (2018), Valencian Medal to Business and Social Impact (2018), Data Scientist of the Year (2019), Jaume I Award in New Technologies (2021) and Abie Technology Leadership Award by AnitaB.org (2021).
Matthew E. Taylor;
University of Alberta & Alberta Machine Intelligence Institute, Canada;
matthew.e.taylor@ualberta.ca
Matthew E. Taylor has worked at multiple academic institutions since his PhD in 2008. He moved to Edmonton in 2017 to lead the Borealis AI lab, the artificial intelligence arm of the Royal Bank of Canada. In 2020 he returned to academia, becoming an Associate Professor of Computing Science at the University of Alberta. He is now also a Fellow-in-Residence at Amii, where he helps bring AI into companies around the world, and also serves as the research director at AI-Redefined, a startup working on human-AI teams in multiagent settings.
Additional Information
For more information visit https://sites.google.com/view/collab-ai-and-human-modeling/home or email us with questions at caihu.aaai2024@gmail.com.
B5: Constraint Programming and Machine Learning
Bringing together Constraint Programming (CP) and Machine Learning (ML) is an important aspect of the larger goal of integrating Reasoning and Learning. Participants are not expected to have prior experience in both fields, but to have familiarity with each at least at the level of an introductory AI course. The Bridge is designed to educate and to build community, to provide opportunities to interact, discuss, raise awareness and find collaborators.
The focus of this one-day Bridge will be on bringing together the traditional AI fields of constraint-based reasoning and machine learning, but participants from related fields of reasoning, optimization and learning, e.g. SAT, operations research, data mining, will be welcome.
Submissions
You can submit in any of a variety of Tracks. There are many Tracks. We do not necessarily expect to receive submissions for every Track, but we wish to maximize opportunities and options for contributing to the Bridge and the Bridge community. You may submit to more than one Track. The simplest option is the Introductions Track, which has minimal requirements, and provides an opportunity for participants to introduce themselves, with a view to facilitating interaction and enabling collaboration, during the Bridge day and afterwards. Note that if we receive too many submissions to this Track to accommodate for physical presentation in the time available, appropriate participants will be chosen for presentation on a first come first served basis, so you are encouraged to submit early.
The full list of Track options is available at the CPML Bridge website, along with full submission requirements and instructions and other important information. Submissions will be through EasyChair.
Dates
- November 24, 2023: Bridge Submissions Due
- December 11, 2023: Notifications Sent to Authors
- December 20, 2023: AAAI Early Registration Deadline
- January 8, 2024: All Materials for Participants Posted
- February 21, 2024: CPML Bridge
Organizers
Eugene C. Freuder,
UCC.
eugene.freuder@insight-centre.org
Professor Freuder is an Emeritus Professor in University College Cork and is affiliated with the Insight Science Foundation Ireland Research Centre for Data Analytics. He received his undergraduate degree from Harvard and his Ph.D. from MIT. He is the recipient of the IJCAI-20 Award for Research Excellence. He was elected a Member of the Royal Irish Academy, and a Fellow of the American Association for the Advancement of Science, the Association for the Advancement of Artificial Intelligence, and the European Association for Artificial Intelligence. He served as a Councilor of the Association for the Advancement of Artificial Intelligence. He has received the Research Excellence Award and the Distinguished Service Award of the Association for Constraint Programming. He was the founding Editor-in-Chief of the Constraints journal, and served as chair of the Organizing Committee of the International Conference on Principles and Practice of Constraint Programming.
Barry O’Sullivan,
UCC.
barry.osullivan@insight-centre.org
Professor Barry O’Sullivan is a professor at University College Cork (UCC) working in the fields of artificial intelligence, constraint programming, operations research, AI/data ethics, and public policy. He contributes to several global Track II AI diplomacy efforts at the interface of geopolitics and artificial intelligence. Professor O’Sullivan is a Fellow and a past President of the European AI Association (EurAI). He is also a Fellow and served as a member of the Executive Council of the Association for the Advancement of Artificial Intelligence (AAAI). In July 2018 Professor O’Sullivan was appointed Vice Chair of the European Commission’s High-Level Expert Group on AI. In 2019 the HLEG-AI published: Ethics Guidelines for Trustworthy AI (April) and Policy & Investment Recommendations for Trustworthy AI (June). In 2019 he became an advisor on AI to the European Commission’s Joint Research Centre. In 2019 Professor O’Sullivan was appointed by Ireland’s Minister for Health to the Health Research Consent Declaration Committee. In 2020 he was appointed Chair of the Oversight Board of Health Data Research UK (North). In 2021 he was appointed by the Minister for Health as Chair of the National Research Ethics Committee for Medical Devices. In 2022 he was appointed by the Minister for Trade Promotion, Digital & Company Regulation to the Enterprise Digital Advisory Forum. His awards include: Fellow of the European AI Association (2012), UCC’s Leadership Award (2013), ACP Distinguished Service Award (2014), Science Foundation Ireland Researcher of the Year (2016), UCC Researcher of the Year (2017), elected to the Royal Irish Academy (2017), Fellow of the Irish Computer Society (2018), Fellow of the Irish Academy of Engineering (2019), IPEC-EATCS Nerode Prize (2020), Science Foundation Ireland Best International Engagement Award (2021), Fellow of the Asia-Pacific AI Association (2022), Fellow of the Association for the Advancement of AI (2022), European AI Association’s Distinguished Service Award (2023).
Steering Committee
- Christian Bessiere (U.Montpellier, LIRMM)
- Luc De Raedt (KU Leuven)
- Eugene C. Freuder (University College Cork)
- Tias Guns (KU Leuven)
- Kevin Leyton-Brown (Uni. of British Columbia)
- Michela Milano (University of Bologna)
- Nina Narodytska (VMware Research, USA) Barry O’Sullivan (University College Cork)
Additional Information
http://osullivan.ucc.ie/CPML2024
B6: Continual Causality
Our bridge proposes to bring together the fields of continual learning and causality. Both fields research complementary aspects of human cognition and are fundamental components of artificial intelligence, if it is to reason and generalize in complex environments. Despite some recent interest in bringing the two fields together, including our AAAI-23 bridge program, it remains unclear how causal models may describe continuous streams of data and, vice versa, for continual learning to exploit learned causal structure.
In this bridge program, we aim to take further steps towards a unified treatment of these fields and to provide the space for learning, discussions, and to connect and build a diverse community. Our bridge will focus on three main objectives: 1) define and react to catastrophic interference and knowledge transfer in learning causal models in the context of a continuous, non-stationary stream of data; 2) understand effective ways for causal structure to aid in leveraging the accumulated knowledge of a continual learning system and interpret distributional shifts; and 3) develop next generation benchmarks that go beyond re-purposing of existing datasets to adequately support the above items and further essential research questions.
Format & Attendance
The bridge activities will span two days and include tutorials on continual learning and causality, several invited vision talks from field leaders, two real-world application sessions to discuss applications of the fields (including their intersection), a panel discussion, oral and poster sessions for accepted short papers, and a closing interactive community discussion session to discuss future challenges and opportunities. Our AAAI-23 bridge enjoyed 30+ participants on average, with a peak of up to 50. We expect the AAAI-24 bridge to be similarly sized, given that there are no requirements to participate.
Submissions
We invite submissions that present either general positions and visions of how to link the two fields, outline challenges that need to be overcome, highlight synergies, and propose tangible future steps or discuss first practical approaches and solutions to relevant problems. Submissions of all papers should be up to four pages (excluding references, and without appendices) in the AAAI format. Submissions will be peer-reviewed using a double-blind review process. The submission deadline is November 24, 2023 (AOE) and more information can be found on our website (https://www.continualcausality.org/cfp/). All submissions will be managed through OpenReview and will later be collected in a Proceedings of Machine Learning Research (PMLR) volume. An overview of last year’s bridge program can be found in this PMLR publication: https://proceedings.mlr.press/v208/mundt23a.html
Bridge Committee
Martin Mundt
Independent Research Group Leader at TU Darmstadt and hessian.AI
martin.mundt@tu-darmstadt.de
Martin Mundt is an independent research group leader at the Technical University of Darmstadt (TU Darmstadt) and the Hessian Center for Artificial Intelligence (hessian.AI), where he leads the Open World Lifelong Learning (OWLL) lab: https://owll-lab.com/. He is also a board member of directors at the non-profit organization ContinualAI, organizer at Queer in AI, and D&I chair of AAAI-2024. Previously, he has obtained a PhD degree in computer science (2021), where his thesis on continual learning has received an award for the best thesis in natural sciences, and an M.Sc. in physics from Goethe University (2015). The main vision behind his research and OWLL is to develop systems that can not only learn continuously, but also successfully recognize novel situations and acquire new data, while autonomously adapting in a robust and interpretable way.
Tyler Hayes
Research Scientist at NAVER LABS Europe tyler.hayes@naverlabs.com
Tyler Hayes is a Research Scientist on the Visual Representation Learning team at NAVER LABS Europe and a Board Member of the ContinualAI Non-Profit Organization. She completed her Ph.D. in Imaging Science at the Rochester Institute of Technology (RIT), advised by Prof. Christopher Kanan. Her research focuses on moving beyond the closed-world train/test paradigm to develop methods capable of lifelong and open-world learning. During her studies, she interned at Facebook AI Research (FAIR) and the U.S. Naval Research Laboratory. Previously, she earned a BS and an MS in Applied Mathematics from RIT.
James Smith
Incoming Senior Research Scientist at Samsung Research America james.smith@continualai.org
James Smith is an incoming Senior Research Scientist at Samsung Research America. His research specializes in lifelong machine learning for computer vision and natural language processing. He is anticipated to receive his PhD in Machine Learning in November 2023 from the School of Interactive Computing at the Georgia Institute of Technology, advised by Dr. Zsolt Kira. Additionally, he serves as a Board Member for the non-profit research organization, ContinualAI.
Keiland Cooper
Candidate at University of California Irvine
kwcooper@uci.edu
Keiland Cooper is a PhD Candidate, cognitive scientist, and neuroscientist at the University of California, Irvine, working with the Fortin lab and many collaborators. He is a co-founder of ContinualAI, conducting artificial intelligence research. He is also a UCI pedagogical fellow and National Science Foundation research fellow.
Devendra Singh Dhami
Assistant Professor at TU Eindhoven and hessian.AI d.s.dhami@tue.nl
Devendra Singh Dhami is Assistant Professor at Eindhoven University of Technology (TU\e). Previously he was a DEPTH research group leader on Causality And neUro-Symbolic artificial intElligence (CAUSE), under The Hessian Center for Artificial Intelligence (hessian.AI) and TU Darmstadt. He received his Ph.D. in Computer Science from University of Texas, Dallas. His current research focuses on causal reasoning with deep learning, putting a special focus on the inference by leveraging tractable circuits. His work has established intricate connections between causality and several research areas in machine learning such as large language models, explainable AI, probabilistic models, adversarial attacks and geometric deep learning. He is also interested in the intersection of causality and neuro-symbolic AI where the causal models inform neuro-symbolic models and vice versa in order to learn better systems.
Adèle Helena Ribeiro
Research Scientist at University of Marburg
adele.ribeiro@uni-marburg.de
Adèle Ribeiro is a Research Scientist in the AI in Biomedicine Lab at the University of Marburg and a visiting researcher at the Heinrich Heine University of Düsseldorf, Germany, Previously, she held a postdoctoral researcher position in the Causal AI Lab, at Columbia University, USA. Her research is centered around advancing the capabilities of machine learning and artificial intelligence tools by incorporating causal and counterfactual reasoning. She is actively working on the development of causal inference and learning tools, with the goal of bridging the gap between causality theory and real-world applications, especially in the realm of the Health Sciences. She received her Ph.D., M.Sc., and B.Sc. degrees from the Institute of Mathematics and Statistics of the University of Sao Paulo (USP), Brazil. For more information, you can visit her academic webpage: https://adele.github.io/
Rebecca Herman
Research Associate at German Aerospace Center rebecca.herman@dlr.de
Rebecca Herman is a Research Associate at the Jakob Runge’s Causal Inference and Climate Informatics Group at the German Aerospace Center. She completed her PhD in Ocean and Climate Physics at Columbia University in the City of New York, advised by Adam Sobel and Yochanan Kushnir. In her PhD she focused on atmospheric dynamics and attribution of Sahel rainfall change, and studied causal inference with Elias Barenboim. She is now focusing on development of causal inference methods and their application to climate science as part of the CausalEarth Project.
Website
https://www.continualcausality.org/
Email: info@continualcausality.org
B8: Exploring the use of Federated Learning for Data-Sensitive applications
Federated Learning (FL) is increasingly important in privacy sensitive domains, such as healthcare, where sharing of private/patient data is a barrier to building models that generalize well in the real world and minimize bias. The aim of this lab is to facilitate education on how to perform Federated Learning on both simulated and real-world studies. Tutorial structure focuses on specific clearly indicated parts for beginners and for more advanced attendees.
Building models that can generalize well in the real world and minimize bias is crucial, and even more so in data-sensitive environments such as healthcare, where inequities with regards to access plague the system across multiple populations. Additionally, sharing data across institutions poses significant privacy, regulatory, and ethical hurdles. Federated Learning (FL) is becoming increasingly important in such cases, and we are hoping that with this tutorial, participants will be able to learn how to design and deploy their models across multiple data silos in an easy manner, while taking multiple various practical issues into consideration.
Topics
- Environment setup (45 minutes), followed by feedback on the process (15 minutes)
- Topic: Getting access to the development environment
- Instructions will be provided publicly at least 2 weeks prior to the tutorial (with an active discussion forum) to enable attendees joining the tutorial session with an already setup environment. On site, we will have people to present and guide attendees that have not been able to set up their environment prior to the session. For those that join the session ready to go, we will have material to engage on related discussions and obtain further feedback to optimize the material for future reference.
- Lecture based introductory material (45 minutes with 15 minutes of Q&A)
- Introduction to Federated Learning (FL)
- Introduction to MLCommons Medical Research Working group (MRWG) [ref], along with its mission, vision, and current technical developments.
- Hands on interactive session (2 hours)
- FL evaluation example through MedPerf:
- Setting up a MedPerf server as a benchmark owner
- Data preparation as a medical data provider for evaluation
- Creation and submission of an ML/DL model as an MLCube container for evaluation
- Retrieval and analysis of results from the MedPerf server as the benchmark owner
- FL training using OpenFL for both different AI workloads while considering numerous considerations, including but not limited to the following:
- Data size across collaborators
- Network delays in sharing model weights
- FL evaluation example through MedPerf:
Format of Bridge
Introductory lectures followed by hands-on session.
Attendance
The target audience of this bridge is intended to be primarily Data Scientists and Computational Scientists (about 20 people in total). Interested parties attending this bridge will be able to adapt their existing centralized algorithms to a federated architecture or build new models. Non-data scientists attending this bridge will learn about both technical and non-technical considerations setting up federations for training medical Al models. Importantly, attendees will also understand the privacy and security attack vectors and mitigations when using Federated Learning.
Submission requirements
We encourage submissions for the poster session on all topics relevant to federated learning and privacy in machine learning via email (fl-tutorials[at]mlcommons[dot]org). Depending on the number of submissions
Bridge Committee
- Spyridon (Spyros) Bakas, Ph.D.
- Patrick Foley, M.Sc.
- Alexandros (Alex) Karargyris, Ph.D.
- Hasan Kassem, M.Eng.
- Sarthak Pati, M.Sc.
Spyridon (Spyros) Bakas, Ph.D
Spyridon (Spyros) Bakas, Ph.D. is an Associate Professor at Indiana University (IU) School of Medicine Department of Pathology and Laboratory Medicine and is the Inaugural Director of the Division of Computational Pathology. He also holds secondary appointments in the Department of Radiology and Imaging Sciences, the Department of Biostatistics and Health Data Science, the Department of Neurological Surgery, and the Department of Computer Science in the Luddy School of Informatics, Computing, and Engineering. Before joining IU, Dr. Bakas was with the Department of Pathology & Laboratory Medicine and the Department of Radiology at the Perelman School of Medicine of the University of Pennsylvania (UPenn), and a secondary affiliation with the Dept. of Bioengineering at the UPenn. Additionally, he is the MLCommons MRWG Vice Chair for Benchmarking & Clinical Translation. You can find his academic profile here: https://medicine.iu.edu/faculty/64865/bakas-spyridon.
Alexandros (Alex)
Karargyris, Ph.D.
Alexandros (Alex) Karargyris, Ph.D. is the MLCommons MRWG Chair. Prior to this, he was a researcher at IBM & NIH for more than 10 years. His research interests are in medical imaging, ML, and mobile health. He has contributed to healthcare commercial products and imaging solutions deployed in under-resourced areas. You can find his profile here: https://www.linkedin.com/in/alexandroskarargyris/
Ezequiel Lanza
Ezequiel Lanza is an AI open source evangelist at Intel. He holds an M.S. in Data Science, and he’s passionate about helping people discover the exciting world of artificial intelligence; Ezequiel is a frequent AI conference presenter and the creator of use cases, tutorials, and guides that help developers adopt open-source AI tools. You can find his profile here: https://www.linkedin.com/in/ezelanza/
Xiaoxiao Li, Ph.D.
Xiaoxiao Li, Ph.D. is an Assistant Professor (tenuretrack) at the Department of Electrical and Computer Engineering (ECE) at the University of British Columbia (UBC) starting August 2021. Before joining UBC, Dr. Li was a Postdoc Research Fellow in the Computer Science Department at Princeton University. Dr. Li obtained her PhD degree from Yale University in 2020. During her PhD studies, she was awarded the Advanced Graduate Leadership Scholarship. Dr. Li is leading the Trusted and Efficient AI (TEA) Lab. Her research interests range across the interdisciplinary files of deep learning and biomedical data analysis, aiming to improve the trustworthiness of AI systems for healthcare. You can find her profile here: https://ece.ubc.ca/xiaoxiao-li/
Hasan Kassem, M.Eng.
Hasan Kassem, M.Eng. is the MLCommons Technical Lead for MedPerf. He is a ML software engineer with a background in MLOps & FL. He holds a Master’s in robotics & intelligent systems, with a focus on FL for Computer Assisted Interventions (CAI). You can find his profile here: https://www.linkedin.com/in/hasan-kassem-02625119b
Sarthak Pati, M.Sc.
Sarthak Pati, M.Sc. is a Software Architect at Indiana University, and is the MLCommons Technical Lead for GaNDLF. He holds a Master of Science in Biomedical Computing (TUM), and works on AI and clinical workflow management, with a focus on AI/ML/DL, and privacy-protected algorithms for healthcare. He has additionally worked towards designing tutorials, training, managing, setting up coding guidelines, etc. for researchers to get a jump start on writing good code using academically proven scientific tools. You can find his academic profile here: https://sarthakpati.github.io/
Bridge External URL
B9: Knowledge-guided Machine Learning: Bridging Scientific Knowledge and AI
Scientific knowledge-guided machine learning (KGML) is an emerging field of research where scientific knowledge is deeply integrated in ML frameworks to produce solutions that are scientifically grounded, explainable, and likely to generalize on out-of-distribution samples even with limited training data. By using both scientific knowledge and data as complementary sources of introduction in the design, training, and evaluation of ML models, KGML seeks a distinct departure from black-box data-only methods and holds great potential for accelerating scientific discovery in a number of disciplines. The goal of our bridge is to nurture the cross-disciplinary community of researchers working at the intersection of AI and science, by providing a common platform to catalyze and cross-fertilize ideas from diverse fields and shape the vision of the rapidly growing field of KGML.
Topics:
We encourage participation on a range of topics exploring the synergy between scientific knowledge and ML, including (but not limited to): (a) use of scientific knowledge as loss functions or hard constraints in the training of ML models for supervised, unsupervised, and semi-supervised applications, (b) design of deep learning architectures that are grounded in scientific theories and generate explainable and physically meaningful feature representations, (c) use of simulated data generated by science-based models along with observations in ML frameworks, (d) techniques to augment imperfections or infer parameters in science-based models using ML, and (e) use of scientific knowledge in the design, pretraining, or finetuning of Foundation models in science.
Format:
Our two-day bridge will include a mix of activities to support education, collaboration, and outreach in the field of KGML including invited talks, lecture-style tutorials, hands-on demos, panel discussions, poster session, and networking/mentoring events.
Submission Requirements:
We are accepting short submissions (maximum 2 pages excluding references) as extended abstracts or proposals in a variety of tracks such as: (a) lecture-style tutorials providing a survey or perspective of a research area in KGML, (b) hands-on tutorials imparting practical understanding of cutting-edge tools and coding platforms in KGML, (c) early career lightning talks promoting next-generation leaders in KGML including graduate students, postdocs, and early career investigators, and (d) posters showcasing new or existing problems, methods, datasets, and evaluation benchmarks in KGML. More details about the submission instructions for every track can be found at our bridge website: https://sites.google.com/vt.edu/kgml-bridge-aaai-24.
All submissions will undergo light review by the organizers for suitability for the bridge.
Submission Site Information:
https://easychair.org/conferences/?conf=kgmlbridgeaaai24
Organizing Committee:
Anuj Karpatne (Virginia Tech, karpatne@vt.edu), Ramakrishnan Kannan (Oak Ridge National Laboratory, kannanr@ornl.gov), and Vipin Kumar (University of Minnesota, kumar001@umn.edu)
Bridge URL:
https://sites.google.com/vt.edu/kgml-bridge-aaai-24
Anuj Karpatne
Virginia Tech
karpatne@vt.edu
Anuj Karpatne is an Associate Professor in the Department of Computer Science at Virginia Tech, where he develops data mining and machine learning methods to solve scientific and socially relevant problems. A key focus of Dr. Karpatne’s research is to advance the field of science-guided machine learning for applications in several domains including climate science, hydrology, ecology, geophysics, trait-based biology, mechanobiology, quantum mechanics, and fluid dynamics. He has received the Outstanding New Assistant Award by the College of Engineering at VT in 2022, the Rising Star Faculty Award by the Department of Computer Science at VT in 2021 and was named the Inaugural Research Fellow by the IS-GEO (Intelligent Systems for Geosciences) Research Coordination Network for 2019. Dr. Karpatne currently serves as the editor-in-chief of the quarterly newsletter SIGAI AI Matters. Dr. Karpatne is also a co-author of the second edition of the textbook, Introduction to Data Mining. He received his Ph.D. in Computer Science at the University of Minnesota in 2017 under the guidance of Prof. Vipin Kumar.
Ramakrishnan Kannan
Oak Ridge National Laboratory kannanr@ornl.gov
Ramakrishnan Kannan is the group leader for Discrete Algorithms at Oak Ridge National Laboratory. His research expertise is in distributed machine learning and graph algorithms on HPC platforms and their application to scientific data with a focus on accelerating scientific discovery by reducing computation time from weeks to seconds. He was the lead for DSNAPSHOT for a COVID-19 project, which is a finalist for the esteemed Association of Computing Machinery’s Gordon Bell Award in 2021, and listing Summit in 3rd place on Graph500 benchmark using the fewest resources; this is the first time an OLCF system has ranked in Graph500. He has been awarded over $2.8M in research funding from various agencies for solving algorithmic problems on HPC platforms. Additionally, he has been the project lead for over $1 million in Department of Defense projects. With over 24 patents issued in USPTO, he was an IBM Master Inventor. He graduated with a Ph.D. under the advice of Professor Haesun Park from Georgia Institute of Technology and M.Sc (Engg) from Indian Institute of Science under the advice of Professor Y. Narahari.
Vipin Kumar
University of Minnesota
kumar001@umn.edu
Vipin Kumar is a Regents Professor at the University of Minnesota, where he holds the William Norris Endowed Chair in the Department of Computer Science and Engineering. Kumar received the B.E. degree in Electronics & Communication Engineering from Indian Institute of Technology Roorkee (formerly, University of Roorkee), India, in 1977, the M.E. degree in Electronics Engineering from Philips International Institute, Eindhoven, Netherlands, in 1979, and the Ph.D. degree in Computer Science from University of Maryland, College Park, in 1982. He also served as the Head of the Computer Science and Engineering Department from 2005 to 2015 and the Director of Army High Performance Computing Research Center (AHPCRC) from 1998 to 2005.
Kumar’s research spans data mining, high-performance computing, and their applications in Climate/Ecosystems and health care. His research has resulted in the development of the concept of isoefficiency metric for evaluating the scalability of parallel algorithms, as well as highly efficient parallel algorithms and software for sparse matrix factorization (PSPASES) and graph partitioning (METIS, ParMetis, hMetis). He has authored over 300 research articles, and has coedited or coauthored 10 books including two text books “Introduction to Parallel Computing” and “Introduction to Data Mining”, that are used world-wide and have been translated into many languages. Kumar’s current major research focus is on bringing the power of big data and machine learning to understand the impact of human induced changes on the Earth and its environment. Kumar served as the Lead PI of a 5-year, $10 Million project, “Understanding Climate Change – A Data Driven Approach”, funded by the NSF’s Expeditions in Computing program that is aimed at pushing the boundaries of computer science research.
Arka Daw
Oak Ridge National Lab
Arka Daw is a Distinguished Staff Fellow (DSF) at Oak Ridge National Lab (ORNL), where he is a member of the Center for Artificial Intelligence Security Research (CAISER). He is also affiliated to the Emerging Cyber Systems Group in the Cyber Resilience and Intelligence Division of the National Security Sciences Directorate. His research primarily focuses on enhancing the generalizability, robustness, and reliability of deep learning models to ensure their safety and trustworthiness. He is also enthusiastic about tackling interdisciplinary challenges in AI for science. Dr. Daw has been an active reviewer for top-tier machine learning conferences/journals such as NeurIPS, ICLR, ICML, AAAI, IJCAI, KDD, SDM, and IEEE-TNNLS. Dr. Daw received his PhD in Computer Science from Virginia Tech, specializing in developing uncertainty quantification techniques for physics-informed machine learning (PIML) models. He also holds a Bachelor’s degree in Electronics and Communications Engineering from Jadavpur University, India.
Nikhil Muralidhar
Stevens Institute of Technology
Nikhil Muralidhar is an Assistant Professor in the CS department at Stevens Institute of Technology. At Stevens, Nikhil directs the Scientific Artificial Intelligence (ScAI) Lab with a research focus on applied machine learning (ML) in domains like physics, fluid dynamics, computational epidemiology, and cyber-physical systems (CPS). The primary research focus at ScAI Lab is to develop machine learning models leveraging data and domain knowledge to model complex real-world processes in scientific and cyber-physical systems.