Published:
2018-02-08
Proceedings:
Proceedings of the AAAI Conference on Artificial Intelligence, 32
Volume
Issue:
Thirty-Second AAAI Conference on Artificial Intelligence 2018
Track:
AAAI Technical Track: Machine Learning
Downloads:
Abstract:
For real-world applications, data are often associated with multiple labels and represented with multiple views. Most existing multi-label learning methods do not sufficiently consider the complementary information among multiple views, leading to unsatisfying performance. To address this issue, we propose a novel approach for multi-view multi-label learning based on matrix factorization to exploit complementarity among different views. Specifically, under the assumption that there exists a common representation across different views, the uncovered latent patterns are enforced to be aligned across different views in kernel spaces. In this way, the latent semantic patterns underlying in data could be well uncovered and this enhances the reasonability of the common representation of multiple views. As a result, the consensus multi-view representation is obtained which encodes the complementarity and consistence of different views in latent semantic space. We provide theoretical guarantee for the strict convexity for our method by properly setting parameters. Empirical evidence shows the clear advantages of our method over the state-of-the-art ones.
DOI:
10.1609/aaai.v32i1.11722
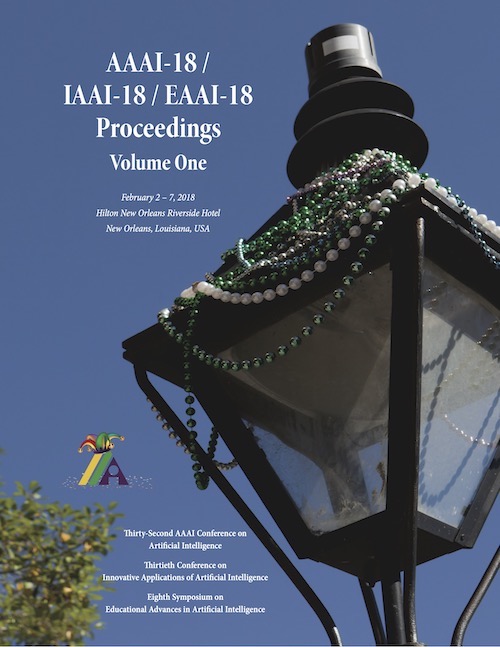
AAAI
Thirty-Second AAAI Conference on Artificial Intelligence 2018
ISSN 2374-3468 (Online) ISSN 2159-5399 (Print)
Published by AAAI Press, Palo Alto, California USA Copyright © 2018, Association for the Advancement of Artificial Intelligence All Rights Reserved.