Published:
2018-02-08
Proceedings:
Proceedings of the AAAI Conference on Artificial Intelligence, 32
Volume
Issue:
Thirty-Second AAAI Conference on Artificial Intelligence 2018
Track:
AAAI Technical Track: Machine Learning
Downloads:
Abstract:
With the advent of multi-view data, multi-view learning (MVL) has become an important research direction in machine learning. It is usually expected that multi-view algorithms can obtain better performance than that of merely using a single view. However, previous researches have pointed out that sometimes the utilization of multiple views may even deteriorate the performance. This will be a stumbling block for the practical use of MVL in real applications, especially for tasks requiring high dependability. Thus, it is eager to design reliable multi-view approaches, such that their performance is never degenerated by exploiting multiple views.This issue is vital but rarely studied. In this paper, we focus on clustering and propose the Reliable Multi-View Clustering (RMVC) method. Based on several candidate multi-view clusterings, RMVC maximizes the worst-case performance gain against the best single view clustering, which is equivalently expressed as no label information available. Specifically, employing the squared χ2 distance for clustering comparison makes the formulation of RMVC easy to solve, and an efficient strategy is proposed for optimization. Theoretically, it can be proved that the performance of RMVC will never be significantly decreased under some assumption. Experimental results on a number of data sets demonstrate that the proposed method can effectively improve the reliability of multi-view clustering.
DOI:
10.1609/aaai.v32i1.11621
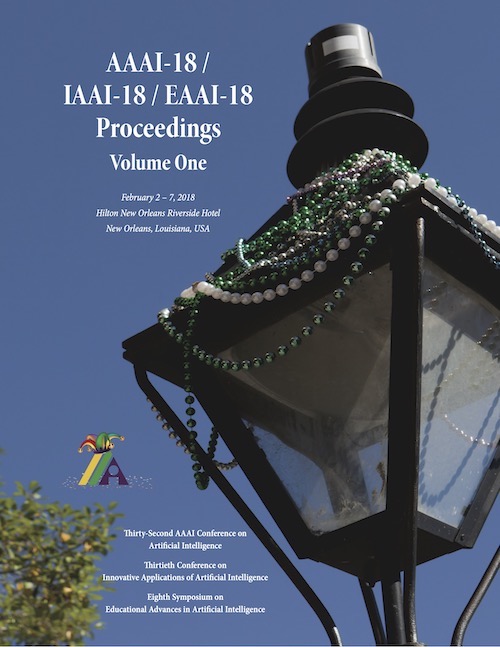
AAAI
Thirty-Second AAAI Conference on Artificial Intelligence 2018
ISSN 2374-3468 (Online) ISSN 2159-5399 (Print)
Published by AAAI Press, Palo Alto, California USA Copyright © 2018, Association for the Advancement of Artificial Intelligence All Rights Reserved.