Published:
2017-10-27
Proceedings:
Proceedings of the AAAI Conference on Human Computation and Crowdsourcing, 5
Volume
Issue:
Vol. 5 (2017): Fifth AAAI Conference on Human Computation and Crowdsourcing
Track:
Full Papers
Downloads:
Abstract:
Structured datasets are difficult to keep up-to-date since the underlying facts evolve over time; curated data about business financials, organizational hierarchies, or drug interactions are constantly changing. Drafty is a platform that enlists visitors of an editable dataset to become ``user-editors'' to help solve this problem. It records and analyzes user-editors' within-page interactions to construct user interest profiles, creating a cyclical feedback mechanism that enables Drafty to target requests for specific corrections from user-editors. To validate the automatically generated user interest profiles, we surveyed participants who performed self-created tasks with Drafty and found their user interest score was 3.2 higher on data they were interested in versus data they had no interest in. Next, a 7-month live experiment compared the efficacy of user-editor corrections depending on whether they were asked to review data that matched their interests. Our findings suggest that user-editors are approximately 3 times more likely to provide accurate corrections for data matching their interest profiles, and about 2 times more likely to provide corrections in the first place.
DOI:
10.1609/hcomp.v5i1.13300
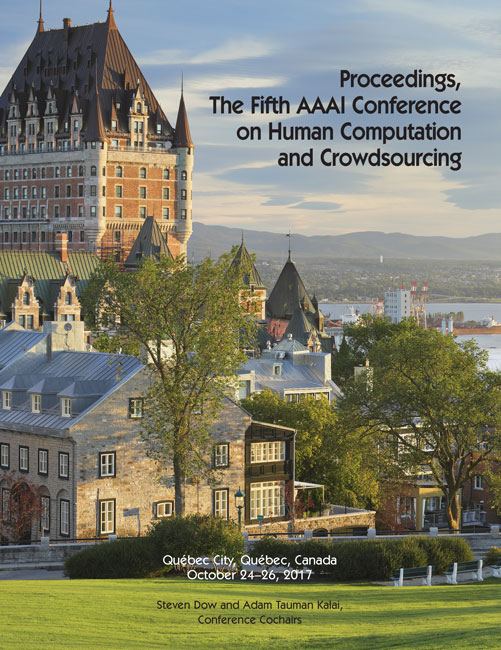
HCOMP
Vol. 5 (2017): Fifth AAAI Conference on Human Computation and Crowdsourcing
ISBN 978-1-57735-793-3