Published:
2017-10-27
Proceedings:
Proceedings of the AAAI Conference on Human Computation and Crowdsourcing, 5
Volume
Issue:
Vol. 5 (2017): Fifth AAAI Conference on Human Computation and Crowdsourcing
Track:
Full Papers
Downloads:
Abstract:
As AI continues to advance, human-AI teams are inevitable. However, progress in AI is routinely measured in isolation, without a human in the loop. It is crucial to benchmark progress in AI, not just in isolation, but also in terms of how it translates to helping humans perform certain tasks, i.e., the performance of human-AI teams. In this work, we design a cooperative game — GuessWhich — to measure human-AI team performance in the specific context of the AI being a visual conversational agent. GuessWhich involves live interaction between the human and the AI. The AI, which we call ALICE, is provided an image which is unseen by the human. Following a brief description of the image, the human questions ALICE about this secret image to identify it from a fixed pool of images. We measure performance of the human-ALICE team by the number of guesses it takes the human to correctly identify the secret image after a fixed number of dialog rounds with ALICE. We compare performance of the human-ALICE teams for two versions of ALICE. Our human studies suggest a counterintuitive trend – that while AI literature shows that one version outperforms the other when paired with an AI questioner bot, we find that this improvement in AI-AI performance does not translate to improved human-AI performance. This suggests a mismatch between benchmarking of AI in isolation and in the context of human-AI teams.
DOI:
10.1609/hcomp.v5i1.13312
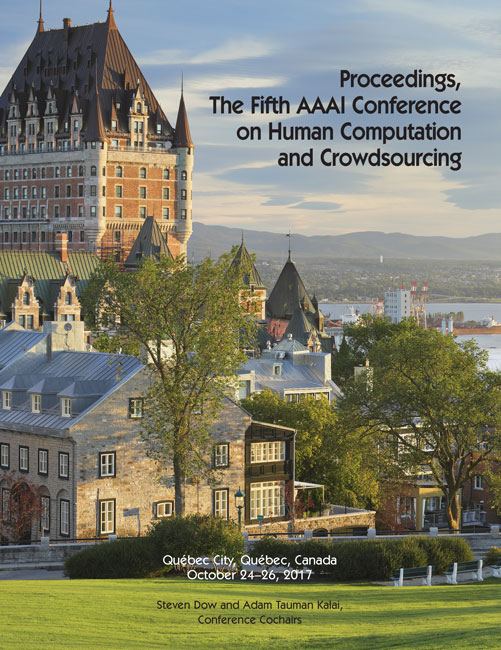
HCOMP
Vol. 5 (2017): Fifth AAAI Conference on Human Computation and Crowdsourcing
ISBN 978-1-57735-793-3