Published:
2014-11-05
Proceedings:
Proceedings of the AAAI Conference on Human Computation and Crowdsourcing, 2
Volume
Issue:
Vol. 2 (2014): Second AAAI Conference on Human Computation and Crowdsourcing
Track:
Works in Progress
Downloads:
Abstract:
Designing pricing mechanisms for recruitment of workers is a central challenge in online crowdsourcing markets. We consider a novel and realistic setting for such markets, where the private cost of the workers and utility derived from them is unknown to the mechanism; however, a set of the workers' features can be observed before making the price offer. How should the offered price be adapted to maximize the utility from recruited workers, while minimizing the cost of payments and the idling cost of failure to recruit? In this paper, we address these questions by formulating the problem as a {em contextual partial monitoring game}, a generic framework for online learning problems that allows to deal with complex feedback structure. We present simulation results comparing our approach to the classical contextual bandit approach, demonstrating the complexity of the problem and the need for the partial monitoring framework.
DOI:
10.1609/hcomp.v2i1.13195
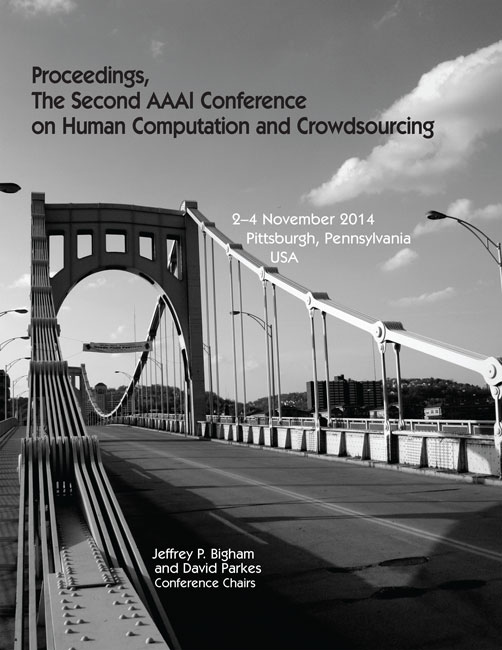
HCOMP
Vol. 2 (2014): Second AAAI Conference on Human Computation and Crowdsourcing
ISBN 978-1-57735-682-0