Published:
2020-06-02
Proceedings:
Proceedings of the AAAI Conference on Artificial Intelligence, 34
Volume
Issue:
Vol. 34 No. 10: Issue 10: AAAI-20 Student Tracks
Track:
Student Abstract Track
Downloads:
Abstract:
To tackle the problem of limited annotated data, semi-supervised learning is attracting attention as an alternative to fully supervised models. Moreover, optimizing a multiple-task model to learn “multiple contexts” can provide better generalizability compared to single-task models. We propose a novel semi-supervised multiple-task model leveraging self-supervision and adversarial training—namely, self-supervised, semi-supervised, multi-context learning (S4MCL)—and apply it to two crucial medical imaging tasks, classification and segmentation. Our experiments on spine X-rays reveal that the S4MCL model significantly outperforms semi-supervised single-task, semi-supervised multi-context, and fully-supervised single-task models, even with a 50% reduction of classification and segmentation labels.
DOI:
10.1609/aaai.v34i10.7179
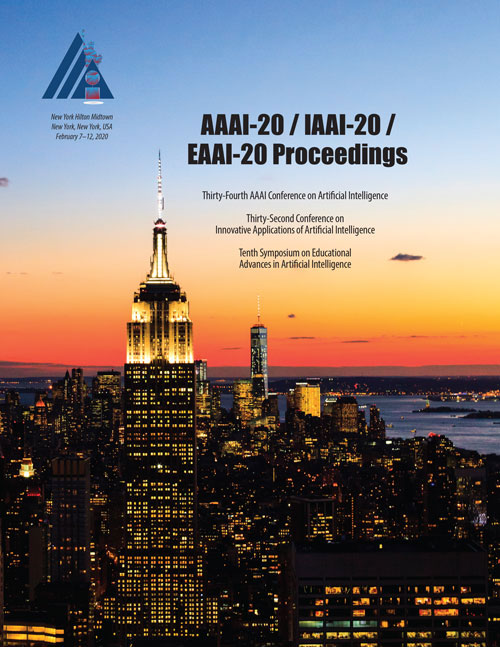
AAAI
Vol. 34 No. 10: Issue 10: AAAI-20 Student Tracks
ISSN 2374-3468 (Online) ISSN 2159-5399 (Print) ISBN 978-1-57735-835-0 (10 issue set)
Published by AAAI Press, Palo Alto, California USA Copyright © 2020, Association for the Advancement of Artificial Intelligence All Rights Reserved