Published:
2020-06-02
Proceedings:
Proceedings of the AAAI Conference on Artificial Intelligence, 34
Volume
Issue:
Vol. 34 No. 01: AAAI-20 Technical Tracks 1
Track:
AAAI Technical Track: Applications
Downloads:
Abstract:
Attributed graphs, where nodes are associated with a rich set of attributes, have been widely used in various domains. Among all the nodes, those with patterns that deviate significantly from others are of particular interest. There are mainly two challenges for anomaly detection. For one thing, we often encounter large graphs with lots of nodes and attributes in the real-life scenario, which requires a scalable algorithm. For another, there are anomalies w.r.t. both the structure and attribute in a mixed manner. The algorithm should identify all of them simultaneously. State-of-art algorithms often fail in some respects. In this paper, we propose the scalable algorithm called MixedAD. Theoretical analysis is provided to prove its superiority. Extensive experiments are also conducted on both synthetic and real-life datasets. Specifically, the results show that MixedAD often achieves the F1 scores greater than those of others by at least 25% and runs at least 10 times faster than the others.
DOI:
10.1609/aaai.v34i01.5482
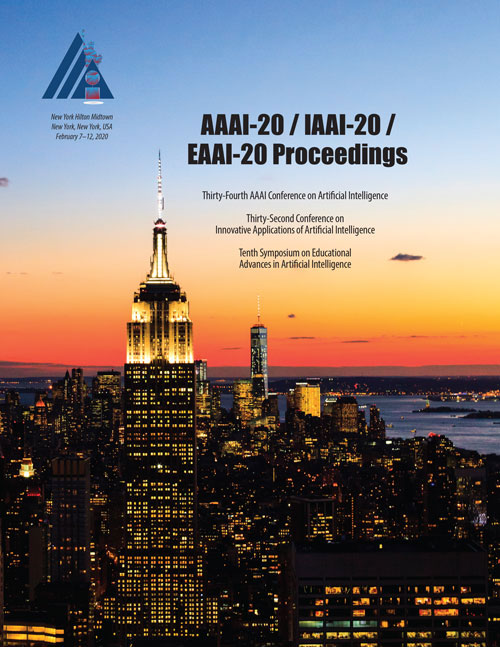
AAAI
Vol. 34 No. 01: AAAI-20 Technical Tracks 1
ISSN 2374-3468 (Online) ISSN 2159-5399 (Print) ISBN 978-1-57735-835-0 (10 issue set)
Published by AAAI Press, Palo Alto, California USA Copyright © 2020, Association for the Advancement of Artificial Intelligence All Rights Reserved