Published:
2020-06-02
Proceedings:
Proceedings of the AAAI Conference on Artificial Intelligence, 34
Volume
Issue:
Vol. 34 No. 01: AAAI-20 Technical Tracks 1
Track:
AAAI Technical Track: Applications
Downloads:
Abstract:
The Kirchhoff law is one of the most widely used physical laws in many engineering principles, e.g., biomedical engineering, electrical engineering, and computer engineering. One challenge of applying the Kirchhoff law to real-world applications at scale lies in the high, if not prohibitive, computational cost to solve a large number of nonlinear equations. Despite recent advances in leveraging a convolutional neural network (CNN) to estimate the solutions of Kirchhoff equations, the low performance is still significantly hindering the broad adoption of CNN-based approaches. This paper proposes a high-performance deep-learning-based approach for Kirchhoff analysis, namely HDK. HDK employs two techniques to improve the performance: (i) early pruning of unqualified input candidates and (ii) parallelization of forward labelling. To retain high accuracy, HDK also applies various optimizations to the data such as randomized augmentation and dimension reduction. Collectively, the aforementioned techniques improve the analysis speed by 8× with accuracy as high as 99.6%.
DOI:
10.1609/aaai.v34i01.5448
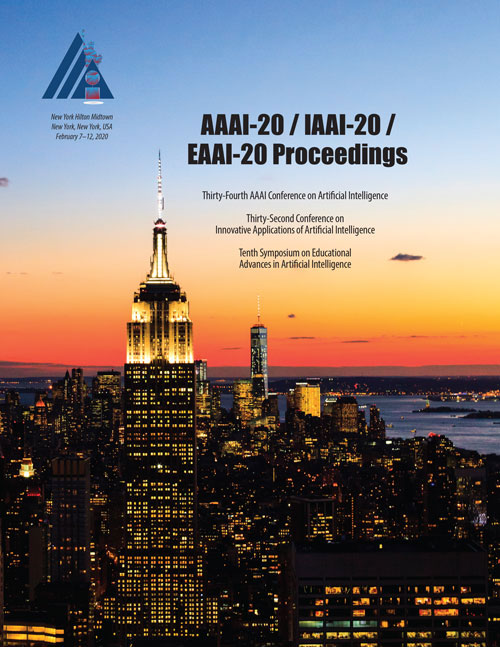
AAAI
Vol. 34 No. 01: AAAI-20 Technical Tracks 1
ISSN 2374-3468 (Online) ISSN 2159-5399 (Print) ISBN 978-1-57735-835-0 (10 issue set)
Published by AAAI Press, Palo Alto, California USA Copyright © 2020, Association for the Advancement of Artificial Intelligence All Rights Reserved