Published:
May 2000
Proceedings:
Proceedings of the Thirteenth International Florida Artificial Intelligence Research Society Conference (FLAIRS 2000)
Volume
Issue:
Proceedings of the Thirteenth International Florida Artificial Intelligence Research Society Conference (FLAIRS 2000)
Track:
All Papers
Downloads:
Abstract:
This paper describes a new framework for using natural selection to evolve Bayesian Networks for use in forecasting time series data. It extends current research by introducing a tree based representation of a candidate Bayesian Network that addresses the problem of model identification and training through the use of natural selection. The framework constructs a modified Naïve Bayesian classifier by searching for relationships within the data that will produce a model for the underlying process generating the time series data. Experimental results are presented that compare forecasts in the presence of multiple sources of information made using the naturally selected belief network versus a random walk.
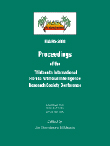
FLAIRS
Proceedings of the Thirteenth International Florida Artificial Intelligence Research Society Conference (FLAIRS 2000)
ISBN 978-1-57735-113-9
Published by The AAAI Press, Menlo Park, California.