Published:
May 2000
Proceedings:
Proceedings of the Thirteenth International Florida Artificial Intelligence Research Society Conference (FLAIRS 2000)
Volume
Issue:
Proceedings of the Thirteenth International Florida Artificial Intelligence Research Society Conference (FLAIRS 2000)
Track:
All Papers
Downloads:
Abstract:
The design of an optimal Bayesian classifier for multiple features is dependent on the estimation of multidimensional joint probability density functions and therefore requires a design sample size that increases exponentially with the number of dimensions. A method was developed that combines classifications from marginal density functions using an additional classifier. Unlike voting methods, this method can select a more appropriate class than the ones selected by the marginal classifiers, thus "overriding" their decisions. For two classes and two features, this method always demonstrates a probability of error no worse than the probability of error of the best marginal classifier.
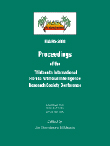
FLAIRS
Proceedings of the Thirteenth International Florida Artificial Intelligence Research Society Conference (FLAIRS 2000)
ISBN 978-1-57735-113-9
Published by The AAAI Press, Menlo Park, California.