Published:
May 2000
Proceedings:
Proceedings of the Thirteenth International Florida Artificial Intelligence Research Society Conference (FLAIRS 2000)
Volume
Issue:
Proceedings of the Thirteenth International Florida Artificial Intelligence Research Society Conference (FLAIRS 2000)
Track:
All Papers
Downloads:
Abstract:
Discrete optimization problems are usually NP hard. The structural characteristics of these problems significantly dictate the solution landscape. In this paper, we explore a structured approach to solving these kinds of problems. We use a reinforcement learning system to adaptively learn the structural characteristics of the problem, hereby decomposing the problem into several subproblems. Based on these structural characteristics, we develop a Genetic Algorithm by using structural operations to recombine these subproblems together to solve the problem. The reinforcement learning system directs the GA. We test our algorithm on the Tactical Fixed Interval Scheduling Problem(TFISP) which is the problem of determining the minimum number of parallel non-identical machine such that a feasible schedule exists for a given set of jobs. This work continues our work in exploiting structure for optimization.
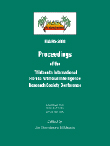
FLAIRS
Proceedings of the Thirteenth International Florida Artificial Intelligence Research Society Conference (FLAIRS 2000)
ISBN 978-1-57735-113-9
Published by The AAAI Press, Menlo Park, California.