Published:
May 1998
Proceedings:
Proceedings of the Eleventh International Florida Artificial Intelligence Research Society Conference (FLAIRS 1998)
Volume
Issue:
Proceedings of the Eleventh International Florida Artificial Intelligence Research Society Conference (FLAIRS 1998)
Track:
All Papers
Downloads:
Abstract:
Relevance reasoning in Bayesian networks can be used to improve efficiency of belief updating algorithms by identifying and pruning those parts of a network that are irrelevant for the computation. Relevance reasoning is based on the graphical property of d {separation and other simple and efficient techniques, the computational complexity of which is usually negligible when compared to the complexity of belief updating in general. This paper describes a belief updating technique based on relevance reasoning that is applicable in practical systems in which observations are interleaved with belief updating. Our technique invalidates the posterior beliefs of those nodes that depend probabilistically on the new evidence and focuses the subsequent belief updating on the invalidated beliefs rather than on all beliefs. Very often observations invalidate only a small fraction of the beliefs and our scheme can then lead to substantial savings in computation. We report results of empirical tests that demonstrate practical significance of our approach.
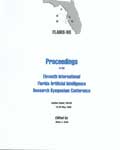
FLAIRS
Proceedings of the Eleventh International Florida Artificial Intelligence Research Society Conference (FLAIRS 1998)
ISBN 978-1-57735-051-4
Published by The AAAI Press, Menlo Park, California