Proceedings:
Proceedings of the AAAI Conference on Artificial Intelligence, 16
Volume
Issue:
Proceedings of the AAAI Conference on Artificial Intelligence, 16
Track:
Student Abstracts
Downloads:
Abstract:
With the recent availability of genome-wide DNA sequence information, biologists are left with the overwhelming task of identifying the biological role of every gene in an organism. Technological advances now provide fast and efficient methods to monitor, on a genomic scale, the patterns of gene expression in response to a stimulus, lending key insight about a gene’s function. With this wealth of information comes the need to organize and analyze the data. One natural approach is to group together genes with similar patterns of expression. Several alternatives have been proposed for both the similarity metric and the clustering algorithm. However, these studies used a specific metric-clustering algorithm pair. In our work, we aim to provide a more systematic investigation into the various metric and clustering algorithm alternatives. We also offer two methods to handle missing data.
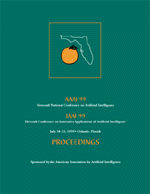
AAAI
Proceedings of the AAAI Conference on Artificial Intelligence, 16
ISBN 978-0-262-51106-3
July 18-22, 1999, Orlando, Florida. Published by The AAAI Press, Menlo Park, California.