Proceedings:
Proceedings of the AAAI Conference on Artificial Intelligence, 16
Volume
Issue:
Proceedings of the AAAI Conference on Artificial Intelligence, 16
Track:
1999 SIGART/AAAI Doctoral Consortium
Downloads:
Abstract:
There are many real world domains where an agent can observe the world state only partially and intermittently, using noisy sensors. Merely keeping track of the objects present in such a system is non-trivial. The problem may be complicated further if the system dynamics are not fully known or unpredictable, so that some on-line learning is necessary. Ihave been working on a principled approach to state estimation and prediction under these realistic conditions. So far, I have focused mostly on object identification, deciding if some newly observed object is the same as a previously observed one. The work has been applied to the surveillance of a large metropolitan freeway system.
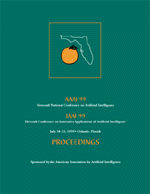
AAAI
Proceedings of the AAAI Conference on Artificial Intelligence, 16
ISBN 978-0-262-51106-3
July 18-22, 1999, Orlando, Florida. Published by The AAAI Press, Menlo Park, California.